Opal wearable sensor device found faster than standard tests in study
New Clario technology may help better track Parkinson's motor symptoms
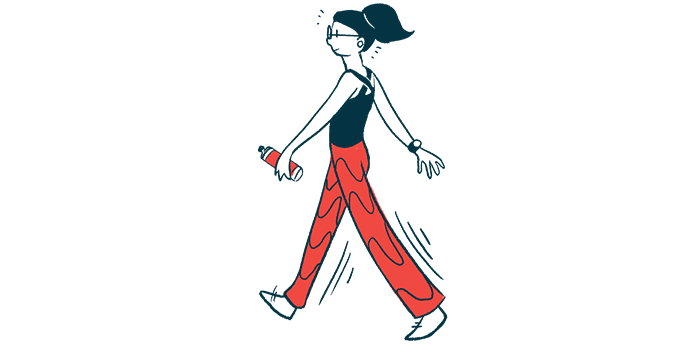
Opal, Clario’s wearable sensor device, was shown to detect early motor progression in people with Parkinson’s disease more rapidly than did standard clinical rating scales.
These findings suggest that this new wearable technology may work better than conventional tests to identify patients with worsening motor symptoms. Such a sensor device also may help speed the development of new Parkinson’s treatments, according to researchers.
The results came from a study led by Chrystalina Antoniades, PhD, of the University of Oxford, who used the device alongside machine learning tools to capture subtle changes in body movements over time. The research was funded by UCB Pharma.
“We are honored to support Professor Antoniades with her pivotal research and are very excited about the positive implications of these results on the Parkinsonism field,” Kristen Sowalsky, PhD, Clario‘s vice president of medical and scientific affairs, said in a company press release.
“This breakthrough demonstrates the essential benefit of using wearable technology and machine learning algorithms to track Parkinson’s disease progression more accurately and assess the efficacy of early therapeutic intervention,” Sowalsky said.
Opal wearable sensor device used to capture data on gait, balance
The hallmark features of Parkinson’s disease include motor symptoms such as tremors, problems with balance and walking (gait), stiffness, and abnormally slow movements. Tests to monitor motor changes are typically conducted by a clinician using various standardized assessments.
However, such evaluations take time, especially for patients whose symptom progression may be less obvious.
Thus, developing new methods to detect subtle body movement changes — and doing so more rapidly than standard tests — may help in speeding disease progression detection. Better tracking technology also could help in therapy efficacy evaluation and the development of treatments to prevent or slow disease progression.
If we can tell whether a treatment is working earlier, we can speed up its translation. It is also important to be able to tell rapidly which things are not working, so we can divert resources to more promising targets.
“Time is crucial in clinical trials,” said Antoniades, an associate professor of neuroscience, and part of the Oxford Quantification in Parkinsonism project, known as OxQUIP.
OxQUIP is following people with Parkinson’s and those with progressive supranuclear palsy, another neurodegenerative condition affecting movement, over two years to identify measures that can detect disease progression over much shorter time periods.
“If we can tell whether a treatment is working earlier, we can speed up its translation. It is also important to be able to tell rapidly which things are not working, so we can divert resources to more promising targets,” Antoniades said.
Opal is a wearable sensor device that detects movements across four body points: the ankles, wrists, lower back, and chest. The device can deliver real-time data on gait, balance, and mobility.
It comes pre-configured with standard assessments embedded in its software that contain more than 140 validated outcome measures. The study to evaluate Opal’s ability to detect motor symptoms changes in Parkinson’s patients was part of the OxQUIP project.
Along with support from Clario’s Precision Motion scientists, the use of the Opal device allowed Antoniades’ team to capture digital outcomes on gait and balance during two standard assessments: the 2-minute walking test (gait) and the 30-second Sway test (balance).
According to Clario, the wearable sensors and machine learning tools were able to track the progression of motor symptoms in Parkinson’s patients with more accuracy — and in significantly shorter timeframes — than standard clinical tests.
“The potential impact of these findings for neuroscience clinical trials is outstanding,” Sowalsky said.