AI Coupled With Videos of Movement May Help in Diagnosing Parkinson’s
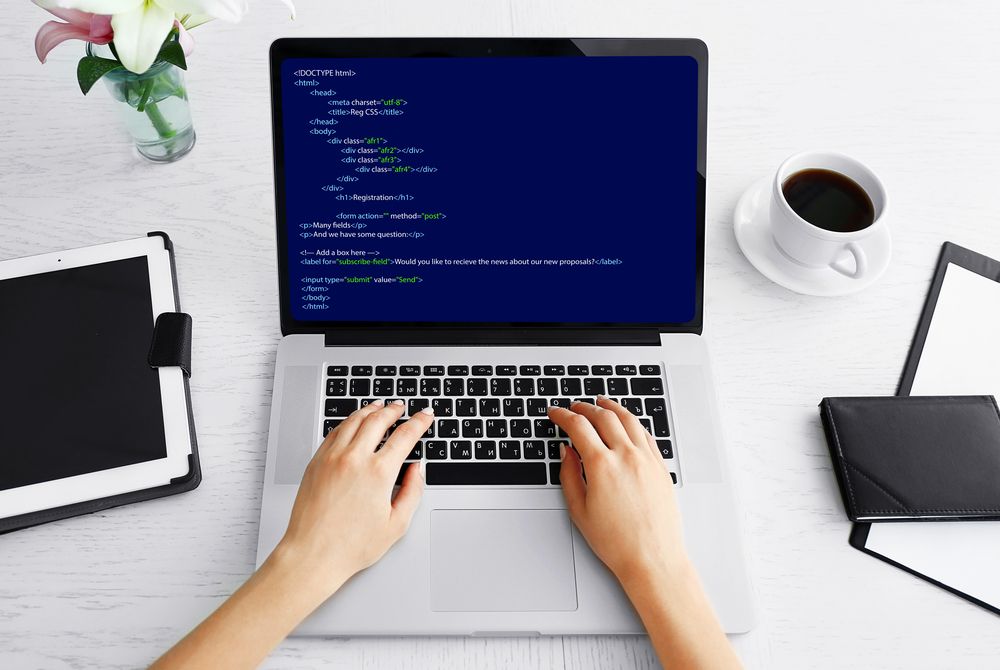
Machine-learning analysis of video recordings of people thought to have Parkinson’s disease as they performed specific motor tasks was able to distinguish these suspected cases from healthy people, as well as Parkinson’s patients from those with essential tremor, a pilot study confirmed.
This non-invasive, artificial intelligence technique has the potential to be a so-called second opinion to help physicians make an accurate Parkinson’s diagnosis more quickly, its researchers said.
The study, “Distinguishing Between Parkinson’s Disease and Essential Tremor Through Video Analytics Using Machine Learning: a Pilot Study,” was published in the IEEE Sensors Journal.
Parkinson’s disease can be challenging to diagnose as there are no specific tests to confirm the disease and many symptoms overlap with those of other neurodegenerative disorders, such as essential tremor — a condition that causes involuntary and rhythmic shaking, similar to Parkinson’s.
The presence of at least two of four symptoms supporting a disease diagnosis includes shaking or tremor, slowness in movement, body rigidity, and difficulty in maintaining balance. The physician may also ask a patient to write, draw, walk, or speak.
Video recording of movements along with computer analysis is a potential non-invasive way to help distinguish between Parkinson’s-related movements and those caused by other disorders, particularly subtle movements that are not visible to the naked eye, especially early in disease progression.
This technique may provide clinicians with an objective “second opinion” to avoid misdiagnosing Parkinson’s, as well as to distinguish between disease stages, monitor the impact of treatments, and recommend patients for deep brain stimulation surgery.
This was the approach taken by researchers at the Skolkovo Institute of Science and Technology in Russia, who built a system that used computer-based, machine-learning algorithms to analyze video recordings of people performing specific movement tasks.
In this pilot study, 83 people with or without neurodegenerative diseases were recorded doing a set of 15 exercises. These included walking, sitting down on a chair, standing up, filling a glass with water, folding a towel, and touching the nose with the index finger. There was also a no movement test to assess tremor at rest, and other activities that clinicians commonly use.
“The exercises were designed under the supervision of neurologists and came from several different sources, including scales that are used for monitoring Parkinson’s disease and previous research done in this area,” Ekaterina Kovalenko, a study co-author, said in a press release.
Videos were then processed using software that places key points onto the body that correspond to joints and the like, creating a simple model of moving subjects to be analyzed by artificial intelligence (machine learning techniques).
Previous research using wearable sensors helped the team detect the most informative exercises for this way of diagnosing Parkinson’s, and ranked these exercises according to how they contributed to a diagnosis.
“Machine learning and computer vision methods we used in this research are already well established in a number of medical applications; they can be trusted, and the diagnostic exercises for Parkinson’s disease have been in development by neurologists for some time,” said Dmitry Dylov, PhD, a study co-author.
“What is truly new about this study is our quantitative ranking of these exercises according to their contribution to a precise and specific final diagnosis. This could only be achieved in collaboration between doctors, mathematicians, and engineers,” Dylov said.
The first classification analysis compared results from healthy participants to those with suspected Parkinson’s, which achieved an f1 score of 0.90 — a measure of a test’s accuracy in which an f1 value of 1 represented a perfect prediction.
A second analysis compared people with an official Parkinson’s diagnosis to those with essential tremor. Here, an f1 score of 0.84 was achieved.
“Our preliminary results show potential in improving diagnosis with the help of video analysis. Our goal is to provide a second opinion for doctors and clinicians, not to replace them,” the scientists wrote. “A video-based method perhaps is the most convenient for patients, as it is the most versatile and non-invasive when compared to various sensors and testing.”
Added Ekaterina Bril, a neurologist and study co-author: “We as doctors see great potential in this; apart from differential diagnosis, we need objective tools to assess motor fluctuation in patients with [Parkinson’s disease]. These tools can provide a more personalized approach to therapy and help make decisions on neurosurgical interventions as well as assess the outcomes of surgery later.”