Using AI to Analyze Gait May Help Diagnose Parkinson’s Disease
Machine learning algorithms also can potentially ID different stages of the illness
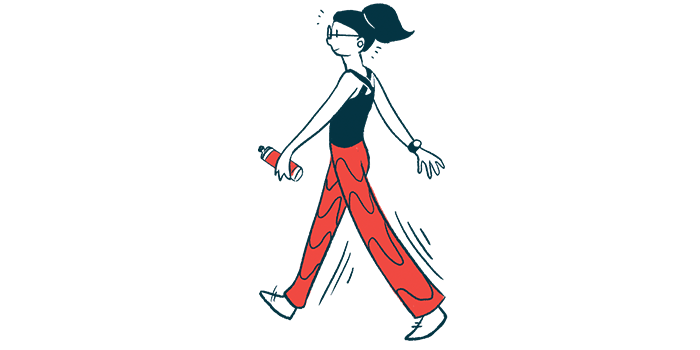
New machine learning models based on walking patterns accurately distinguished between Parkinson’s patients and healthy people, and between different stages of the disease, a study showed.
“We chose gait parameters as the key criteria because gait impairments appear early in Parkinson’s and get worse over time, and also because they don’t correlate with physiological parameters like age, height, and weight,” said Fabio Augusto Barbieri, PhD, one of the study’s authors and a professor at São Paulo State University (UNESP)’s School of Sciences, in Brazil, in a press release.
The study, “Machine learning models for Parkinson’s disease detection and stage classification based on spatial-temporal gait parameters,” was published in Gait & Posture.
There is no test to diagnose Parkinson’s disease. One is made based on characteristic symptoms and signs, which can cause challenges and delays, especially in the early stages of the disease. This has resulted in efforts to identify new, objective measures that can improve the diagnostic accuracy for Parkinson’s.
“Gait analysis is an interesting option with easy interpretation to be considered in PD [Parkinson’s disease] diagnostic and follow up, mainly, due to gait impairments appearing early in PD and getting worse over time, differentiating the PD stages,” the researchers wrote.
Machine learning to diagnose, distinguish disease stages
Barbieri’s team, along with colleagues in Portugal, tested whether machine learning could diagnose Parkinson’s and distinguish between disease stages based on analyses of walking patterns. Machine learning is a form of artificial intelligence that uses algorithms to analyze data, learn from its analyses, and then make a prediction about something.
The researchers recruited 63 people (34 men and 29 women; mean age 68.7) diagnosed with Parkinson’s alongside an equal number of healthy people (18 men and 45 women; mean age 68). Both groups were matched in terms of age, height, and body mass.
All completed an assessment that had them walk three times at a self-selected pace for 8.5 meters (about 27.89 feet) on an unobstructed walkway while wearing markers on their feet and being filmed with specialized cameras. The footage was used to calculate various parameters related to their gait, such as the length of their steps.
The team then used the gait data to “train” machine learning algorithms. As is common practice for these types of studies, the algorithms were trained on a subset of the data and then the accuracy of the “learned” strategies was tested using the remaining data.
The team tested various machine learning algorithms. The best performing, called Naïve Bayes, could distinguish between people with or without Parkinson’s at an accuracy of 84.6% based on four parameters: step length, step velocity, step width, and step width variability, or how much difference there is in step width between one step and the next).
The results showed that the studied [machine learning] algorithms have potential both to PD diagnosis and stage identification by analyzing gait parameters.
Matters of accuracy
“Typical accuracy for [diagnosis based on] clinical assessments is around 80%. We could significantly reduce the probability of diagnostic error by combining clinical assessment with artificial intelligence,” Barbieri said.
Among the patients, 42 had mild disease and the other 21 had moderate disease, as assessed by standard staging systems. In further tests, the researchers used gait-based machine learning algorithms to sort between these two levels of disease severity.
To test the accuracy of this sorting, the team calculated a statistical measure called area under the curve (AUC), which tests how well a given test can distinguish between two groups. AUC values can range from 0 to 1, with higher values indicating a better ability to differentiate.
The best-performing algorithm, called Random Forest, had an AUC value of 0.786. Each of the analyzed algorithms “chose the optimum number of two features, which include stride width variability and step double support time variability,” the team wrote. Double support time is the time during which both feet are in contact with the ground.
“The results showed that the studied [machine learning] algorithms have potential both to PD diagnosis and stage identification by analyzing gait parameters,” the scientists said, adding more research with more participants is needed to confirm these findings, to optimize these models by combining gait pattern data with motor and/or non-motor symptoms and to explore low-cost systems to acquire the data. The equipment used to monitor gait in this study is expensive, which could limit access to this type of testing, they noted.
“We made progress with the tool and contributed to expansion of the database, but we used expensive equipment that’s hard to find in clinics and doctor’s offices,” said Tiago Penedo, one of the study’s authors and a PhD candidate at Barbieri’s lab. “It’s possible to analyze gait with cheaper techniques, using a chronometer, force plate and so on, but the results aren’t precise.”