Digital Home Sensors May Help With Early Detection of Parkinson’s
Non-contact sensors can identify early changes in movement and behaviors
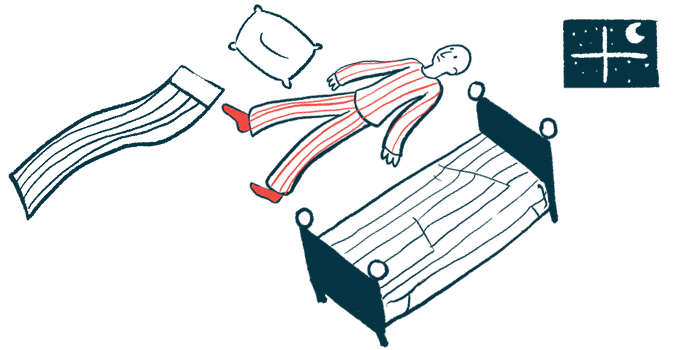
In-home, non-contact sensors that can identify changes in movement and behaviors may allow age-related diseases, including Parkinson’s, to be detected early, a study reports.
By using machine learning tools, the study shows how outcomes such as fall risk, frailty, and cognitive abilities may be assessed in a home setting. Such data may help scientists to discover new digital biomarkers to monitor disease activity.
“We were able to show that such a systems approach — in contrast to the common use of a few health metrics — [helps] to detect age-relevant health problems such as cognitive impairment, fall risk or frailty surprisingly well,” Tobias Nef, PhD, co-study lead, from the University of Bern, in Switzerland, said in a press release.
The study, “A systems approach towards remote health-monitoring in older adults: Introducing a zero-interaction digital exhaust,” was published in the journal npj Digital Medicine.
The four hallmark motor symptoms of Parkinson’s disease include resting tremor, rigidity, postural instability, and slowness of movement (bradykinesia). Many patients also develop non-motor symptoms, such as fatigue, depression and anxiety, sleeping problems, and cognitive changes.
Detecting changes in body movements and behavior may help identify people with Parkinson’s, especially in the early stages of the disease.
Sensor-derived digital health measures have been used to monitor the health status of users. Although mobile devices are an effective way to collect health-related data, they may not be ideal for long-term monitoring in older adults, who tend to be more wary of new technologies. Older adults also have reduced compliance with long-term monitoring, and experience motor and memory problems that make wearing and maintaining devices difficult.
Sensors can help create digital health-related profile based on daily activity
An alternative method, used by the University of Bern research team, was a large-scale, contactless, sensor-based health monitoring approach in the homes of older adults. This approach can create a digital health-related profile based on daily activity and behavior patterns measured by sensors.
“We used non-contact sensors at home to create an extensive collection of digital measures that capture broad parts of daily life, behavior and physiology, in order to identify health risks of older people at an early stage,” said Narayan Schütz, PhD, the study’s first author.
The sensors did not record sound or video for privacy reasons, and their installation was voluntary.
“To ensure privacy and data protection on a technical level, the highest Swiss and European medical data security standards are applied,” Schütz added.
Real-world remote monitoring included two groups of older Swiss adults, with a mean age of 87, of whom 67% were female. Over one year, the team collected 1,268 health parameters from sensors that monitored movement and activity in each room, under mattresses, and on the front and refrigerator doors.
Machine learning tools analyzed the information to derive digital clinical outcome assessments and automatically classifed aging-related health outcomes. Five datasets were created based on fall risk, frailty, late-life depression, and mild cognitive impairment.
At the same time, all participants underwent standard clinical assessments, such as the timed up-and-go test (TUG) to assess fall risk, in which individuals stand up, walk, turn, walk back, and sit. Also included were the Tinneti performance-oriented mobility assessment (POMA) to measure balance and gait; the Edmonton frail scale (EFS) to gauge frailty among older adults; the geriatric depression scale (GDS); and the Montreal cognitive assessment (MoCA).
Based on sensor information, the highest discriminative power, or the ability to identify risk, was achieved with the POMA-based fall-risk dataset when both demographic and digital-derived information were included. Here, an AUC of 0.805 was reached.
The AUC (or Area Under the Curve) is a measure of the ability of a classifier to distinguish between classes. The higher the AUC, the better the model is able to distinguish between positive and negative classes. An AUC of 0.5 indicates no discrimination, 0.7 to 0.8 is acceptable, and 0.8 to 0.9 is excellent. In this case, however, demographic information alone was sufficient to generate good performance (AUC = 0.777).
Performance on other tests was also high, including the TUG with an AUC of 0.786, cognitive-related MoCA with an AUC of 0.780, and frailty-related EFS with an AUC of 0.704. The worst performance occurred with the dataset based on the depression-related GDS, with an AUC of 0.620.
The team noted that adding digital information resulted in significantly higher AUC values than those obtained with demographic information alone, except for the POMA and GDS datasets.
Data shows poor sleep quality is linked to higher fall risk
The importance of each digital measure, that may be used as a digital biomarker, was then evaluated. In the fall-risk outcome based on the TUG dataset, high overall in-bed activity, such as restlessness or poor sleep quality, was related to higher fall risk.
“We found indications that fall risk could significantly depend on certain sleep parameters,” said Nef.
Based on POMA data, older adults with more movement in the home had a lower fall risk, and older adults who open the refrigerator later in the day may be frailer. In addition, those that tend to spend more time in bed may reflect worse depressive symptoms, and adults with mild cognitive impairment appear to have more variation in nightly heart rate dipping.
“We introduce a comprehensive set of digital measures, what may be referred to as a digital exhaust, for long-term remote health-monitoring in the older adult demographic,” the researchers wrote. “We show how ageing-relevant outcomes such as fall risk, frailty, and [mild cognitive impairment] may be assessed.”
Last, “we highlight the possibility of using the digital exhaust to discover novel digital biomarker candidates,” the researchers added.
Hugo Saner, PhD, the study’s co-lead, said: “Such a system marks a milestone in early detection of worsening health for seniors living alone into old age. We assume that it can make a significant contribution to enabling older people to live at home for as long as possible by delaying hospital admissions and transfers to nursing institutions or, in the best case, even avoiding them.”