Fat levels in blood may help predict Parkinson’s symptoms, study finds
Machine learning algorithm can predict long-term symptom outcomes
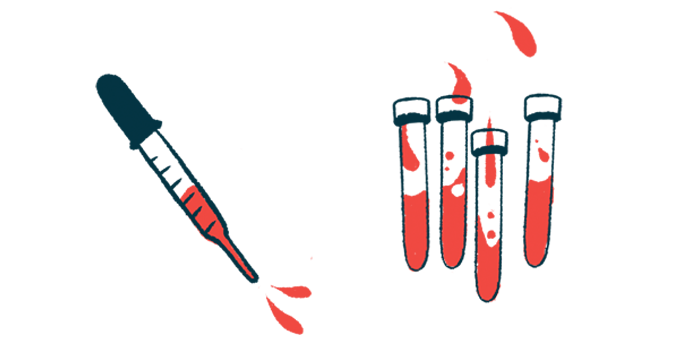
Measuring levels of lipids (fat molecules) in the blood may help predict the severity of symptoms in Parkinson’s disease, a new study suggests.
The study, “Prediction of motor and non-motor Parkinson’s disease symptoms using serum lipidomics and machine learning: a 2-year study,” was published in npj Parkinson’s Disease.
Parkinson’s affects every person with the disease differently, and it can be difficult to predict how the disease will progress for any individual. While the causes of Parkinson’s aren’t fully understood, several studies have suggested the disease is characterized by abnormalities in lipid levels, which may play a role in disease progression.
A team of scientists in Australia set out to determine whether analyzing blood lipid levels might help predict the severity of Parkinson’s symptoms. The team first conducted detailed assessments of more than 900 different lipids in 122 people living with Parkinson’s. Then, they collected standardized assessments of symptom severity over the course of two years for 67 of these patients.
Machine learning models developed to predict symptoms based on lipid profiles
With these data in hand, the researchers created machine learning models to predict symptom scores based on lipid profiles. In machine learning, a computer is fed a large dataset along with a set of mathematical rules called algorithms, which the computer uses to “learn” and find patterns in the data.
Results demonstrated the machine learning algorithm trained on lipid profiles, as well as clinical and demographic data, was able to predict long-term symptom outcomes accurately, with particularly good predictive power for measuring the severity of motor symptoms and of depression, a common nonmotor symptom of Parkinson’s.
Several specific types of lipids that were shown to be especially good at predicting symptom outcomes have previously been implicated in Parkinson’s biology, the researchers noted. These data “generated a shortlist of serum lipid candidates that may have a role in the progression of [Parkinson’s],” they said, highlighting potential avenues for future investigations.
The lipids-based machine learning models were notably better at predicting long-term symptom outcomes than similar models that were trained on a panel of cytokines (inflammatory signaling molecules). In the models, the lipid profile was more important for making accurate predictions than demographic factors such as age and sex, and also more important than status of mutations in the LRRK2 gene, a well-established genetic risk factor for Parkinson’s.
The scientists stressed this analysis was limited to a fairly small number of patients followed for a relatively brief time, so additional work will be needed to validate and expand on these results.
“Assessment of predictor robustness is necessary and a longer follow-up, larger cohort and more diverse clinical scores are advised to predict these scales in the future,” the team wrote.