Wearable sensors may help to quickly spot patients at risk of falls
Data captured in gait, posture tests ID'd those with falls over next few years
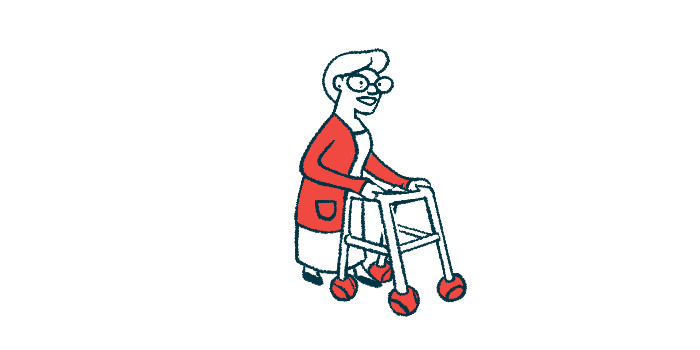
Data from a brief gait and posture assessment using wearable sensors — taking just a few minutes in the clinic — could help to accurately predict the risk of falls in the near future with Parkinson’s disease, a recent clinical study reported.
Scientists at the University of Oxford in England tested the method in more than 100 Parkinson’s patients without previous falls. An artificial intelligence (AI)-powered predictive model based on sensor data accurately distinguished patients who went on to have falls over the next two and five years from those who didn’t.
This study “contributes to the understanding of the risk of falls in [Parkinson’s disease] showing that wearable [sensors] and machine learning can be used to identify potential fallers … from a single gait and posture data collection session lasting less than [three minutes],” the researchers wrote.
The study, “Predicting future fallers in Parkinson’s disease using kinematic data over a period of 5 years,” was published in npj Digital Medicine.
Known fall risk factors include previous falls and changes in gait and posture
Parkinson’s patients experience a range of disease motor symptoms that can impair their walking abilities and increase their risk of falling. In turn, frequent falls can lead to injuries as well as anxiety and fear, affecting their quality of life.
Known risk factors include a history of previous falls, as well as aspects of posture and gait. But clinical assessments of gait slowing or variability and postural instability using traditional approaches can be subjective and time consuming.
Still, it’s important to understand who is at a risk of falls in order to effectively come up with a care plan to avoid them.
“A more objective way to prospectively identify future fallers before their first fall is desirable,” the researchers wrote.
Increasingly, it has been suggested that wearable sensors are a promising way for identifying Parkinson’s and monitoring the disease. Such sensors can collect large amounts of information about how a person moves, which is then analyzed using computer algorithms to make predictions.
Scientists at Oxford investigated whether a very short sensor-based assessment in the clinic could predict falls over a five-year period among 104 Parkinson’s patients, all participating in an ongoing and long-term university study called the Oxford Quantification in Parkinsonism. None had a prior history of falls.
At the clinic, participants carried out quick, physical tasks while wearing six sensors on their chest, waist, wrists, and feet.
Specifically, they performed the 2-minute walk test — tracking distance walked over that time on a straight, level surface — as well as a 30-second postural sway task, where people stand still with their eyes closed and body movement is measured.
Shorter stride lengths recorded in Parkinson’s patients with a fall risk
Patients had follow-up visits two and five years later to determine if they had fallen. At two years later, 13 people had falls, and 23 people reported falling by year five.
Analysis of AI algorithms, used to study the sensor data and develop models for predicting falls, revealed several significant differences in walking and postural features between patients with and without falls.
Predictive models generated from that data showed a strong ability to predict falls after two years, with an accuracy of 84%-92%. The most accurate model after five years, which accounted for selected sensor features as well as demographic data like a person’s age, had a predictive accuracy of 78%.
“Mean stride length was found to be an important predictor of future falls,” the researchers wrote, adding that the “decrease in stride length observed in the participants at risk … may suggest a compensatory mechanism to manage deteriorating dynamic balance.”
During the study, the researchers also collected information from commonly used questionnaires and clinical scales.
Data here showed that patients who went on to fall were older and had been living with Parkinson’s for longer. They generally also had poorer motor function, mobility, and self-reported disability.
“Our study presents a digital approach to predicting first falls in patients with [Parkinson’s disease] using wearable sensor data,” the researchers wrote, adding that such data could help doctors in targeting prevention strategies to those most at risk.
“The natural next step, if we are able to predict the risk of falls, is to offer a medical intervention to prevent their occurrence,” they wrote.
A number of different interventions, from exercise to a review of current medications, have been proposed for that purpose. The use of the sensor data could help in identifying patient groups most likely to benefit from such programs and interventions, according to the scientists.