Symptom-responding deep brain stimulation system in development
Researchers are using neuromorphic computing to improve on open-loop DBS devices
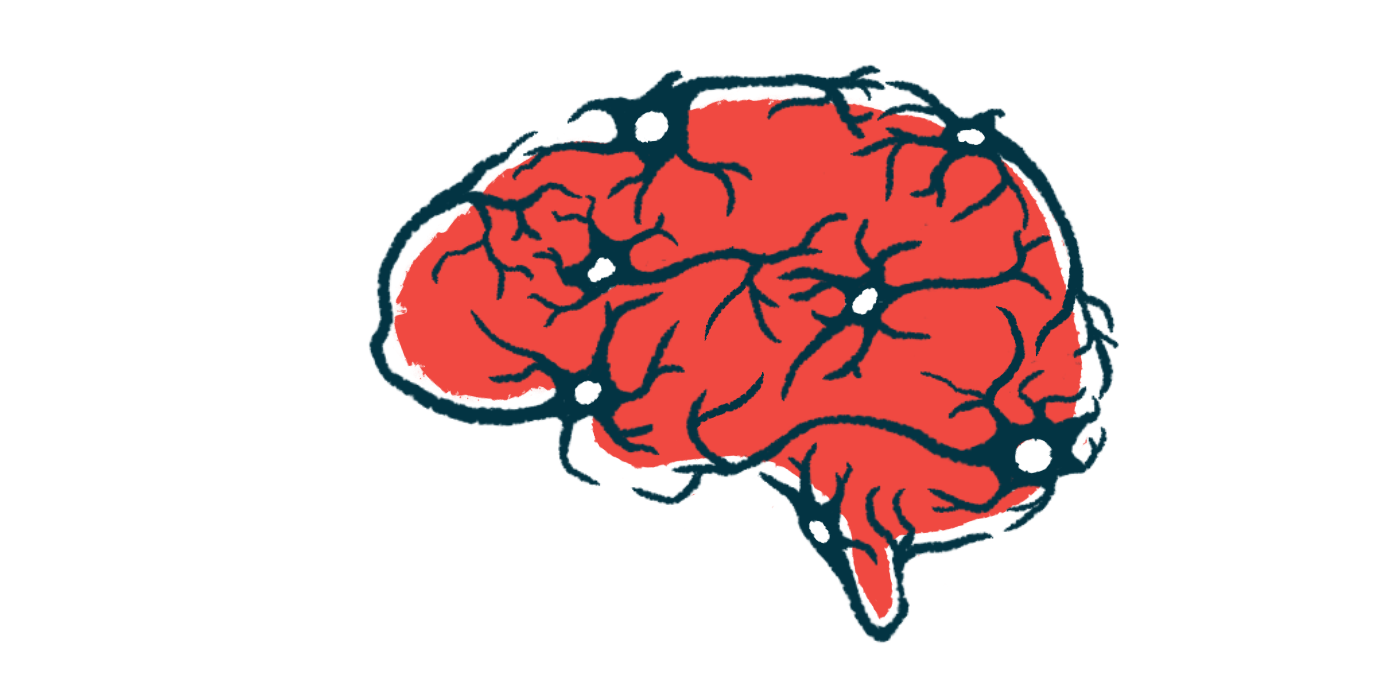
Researchers at Michigan Technological University are developing a “smart” deep brain stimulation (DBS) system to treat Parkinson’s disease that’s automatically activated only when needed, making it more effective and energy-efficient.
The researchers are using neuromorphic computing — an approach inspired by the structure and function of the human brain — to improve on current open-loop DBS devices that generate constant stimulation, which can lead to unwanted side effects.
The new closed-loop system has the potential to adjust stimulation signals according to the severity of a patient’s symptoms. This could mean increased effectiveness and fewer side effects, along with less energy consumption, meaning fewer surgeries to replace batteries.
“Exploiting neuromorphic computing to improve deep brain stimulation for Parkinson’s disease is very innovative,” Chunxiu (Traci) Yu, PhD, the project’s co-lead and an assistant professor of biomedical engineering at Michigan Technological University, said in a university news release. “To our knowledge, this is the first effort in the field.”
DBS is an alternative to Parkinson’s medications after they lose effectiveness over time. Small wires are surgically implanted in specific areas of the brain to stimulate them with electrical impulses and reduce motor symptoms such as tremors, muscle stiffness, and slowed movements.
The wires are connected to a battery-powered pulse generator surgically inserted in the chest or abdomen. Once implanted, stimulation settings can be adjusted according to the patient’s needs.
However, “most current DBS systems are open-loop,” Yu said, meaning signals are sent constantly regardless of the presence and/or severity of symptoms “because the real-time symptoms are unknown to the device.”
Besides the potential side effects caused by continuous stimulation, being “on 24 hours a day, 365 days a year” makes them “high in energy consumption,” Yu said. This means surgeries are needed every two to five years to replace the device’s batteries.
Making deep brain stimulation better for Parkinson’s patients
Yu and colleague Hongyu An, PhD, a Michigan Tech assistant professor of electrical and computer engineering, are applying neuromorphic computing in an effort to improve DBS.
“Referred to as brain-inspired computing or neuroscience-powered artificial intelligence, neuromorphic computing emulates a nervous system using microchips and algorithms,” Yu said. “It is also highly energy-efficient.”
Together, Yu, An, and their teams are developing a closed-loop DBS system “that can intelligently adjust stimulus signals according to patient symptoms.”
“Using a closed-loop system allows us to optimize the energy efficiency of DBS devices,” Yu said, adding “the patient’s brain signals are used to generate a treatment signal — a stimulation — as needed, in real time.”
At the heart of the closed-loop DBS are spiking neural networks (SNNs), a type of artificial neural network that, much like natural neural networks, transmit information only once a spike threshold has been reached.
“The communication signals within SNNs are represented with small spike electrical pulses in volts,” An said, adding, because SNNs incorporate the concept of time, like intervals between spikes, “SNN systems have much higher energy efficiency compared to other artificial neural networks.”
The new system detects beta oscillations, the type of brain waves generated by brain regions that control body movements. By measuring brain activity there, it can evaluate motor symptoms’ severity.
“We use the beta oscillatory activity as a biomarker because it can be detected much faster than other means, such as tremor signals,” An said. “If the neural activity detected is unusually strong, it indicates the Parkinson’s disease symptoms are more severe.”
The teams have been using Loihi, Intel’s neuromorphic chip, which “outperform other computational platforms in terms of energy efficiency by 109 times,” An said.
Another innovative feature of the new closed-loop DBS system is the replacement of SNN’s traditional electronic memory for a memristor. Besides remembering the amount of charge that flowed through it, letting it store information like a memory chip, memristors can control the flow of electrical current, like a resistor.
Unlike standard resistors though, “the resistance of a memristor can be changed into multiple or even thousands of different resistances,” An said. “This feature significantly increases the amount of information that can be stored by individual memristors.”
According to data presented last year at the 23rd International Symposium on Quality Electronic Design, the teams’ simulations using SNNs and memristors reduced the size of chips by 67.3% and generated 41.9% faster signal transmission while using 11.7% less energy.
“This result is highly promising,” An said.
The next goal is to design their own memristor neuromorphic chip customized to the closed-loop DBS.
“Our research on these new, innovative computational paradigms — along with the design of emergent AI chips — will open a new door to greater and faster development of smart medical devices for brain rehabilitation,” An said. “Even wearable medical devices are now well within the realm of possibility.”