Parkinson’s Disease Digital Biomarker DREAM Challenge Winners Announced
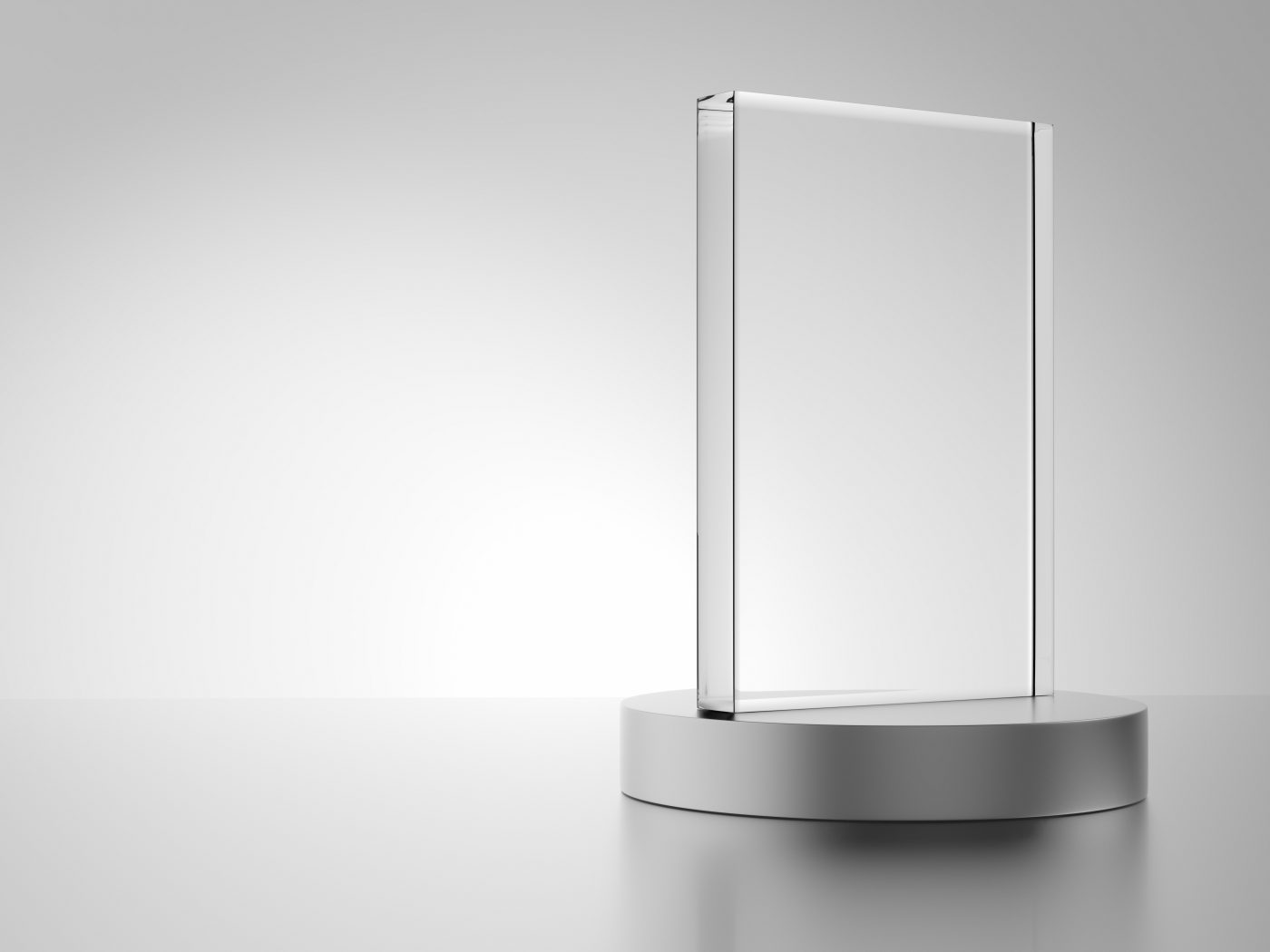
The winners of a crowd-sourced research challenge designed to improve the ability of remote sensors to monitor Parkinson’s disease (PD) used a mix of signal processing and deep neural networks to better predict disease and disease severity.
Sage Bionetworks, in collaboration with the Michael J. Fox Foundation, recently published the results of the Parkinson’s Disease Digital Biomarker (PDDM) DREAM Challenge. The methods developed by top-performing teams performed 38 percent better than previous models at detecting Parkinson’s from a walk and balance test, and were 58 percent better than baseline models at predicting severity of different symptoms, among other achievements.
“The proposed solutions were far outside the traditional techniques used in the field of actigraphy (a sensor used to measure gross motor activity) and many of the experts involved in organizing the challenge are reconsidering the way they interpret this kind of data,” Larsson Omberg, vice president of systems biology at Sage Bionetworks, said in a press release.
The PDDM DREAM Challenge was divided into two categories. Participants in the first category used data from mPower (a large health study where Parkinson’s patients used their mobile phones to perform walk and balance tests) to extract features that could be used to detect the disease. In the second category, participants extracted features for three different symptoms (tremor, dyskinesia and bradykinesia) from a Michael J. Fox Foundation-funded study, the Levodopa Response Trial (where people were monitored with three to eight accelerometer sensors while performing a series of activities).
Yuanfang Guan and Marlena Duda from the University of Michigan, Ann Arbor, won the first category. The team developed a deep learning convolutional neural network with artificial intelligence technology that led to a predictive model identifying Parkinson’s 38 percent better than baseline models.
The three award winners in the second category were Bálint Ármin Pataki, from Eötvös Loránd University in Hungary; Jennifer Schaff, a Data Scientist at Elder Research, Inc.; and Yuanjia Wang and Ming Sun, from Columbia University. Pataki built features for tremor severity that performed 11 percent better than previous models. Schaff developed statistical methods to derive features that predicted dyskinesia severity 59 percent better than baseline models. Wang and Sun built features using spectral decomposition that outperformed all other teams in predicting bradykinesia with a 17 percent improvement.
The next step is moving participants into a joint effort to learn from each other’s experiences and work together to find new methods that might improve the value of the new findings and help interpret the clinical relevance of new features found during the challenge.
More than 440 data experts participated in the challenge from all corners of the world. The DREAM Challenge is funded by the Michael J. Fox Foundation and the Robert Wood Johnson Foundation.