Machine learning tool may help detect Parkinson’s disease: Study
Proposed model uses EEG data to analyze brain activity over time
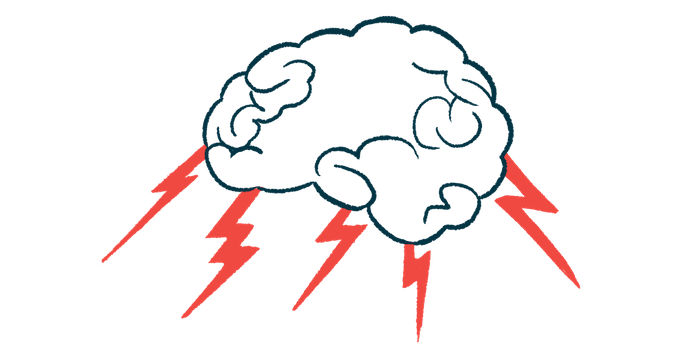
A new machine learning model is able to identify Parkinson’s disease more accurately than other models based on electroencephalography (EEG) readings of the brain’s electrical activity, a study found.
“The proposed model holds promising potential as a valuable and enduring aid for experts and clinicians in diagnosing” Parkinson’s, researchers wrote in the study, “An efficient Parkinson’s disease detection framework: Leveraging time-frequency representation and AlexNet convolutional neural network,” which was published in Computers in Biology and Medicine.
There is no test that can conclusively diagnose Parkinson’s disease. Instead, diagnosis is made based on evaluation of the patient’s symptoms and elimination of other possible causes. This is often a time-consuming and frustrating process, and a major goal of Parkinson’s research is to find faster and more reliable ways to diagnose the disease.
EEG, a tool that measures electrical activity in the brain via electrodes placed on the scalp, is frequently used in the diagnostic workup for people with Parkinson’s. Several studies in recent years have explored using machine learning algorithms to analyze EEG in hopes of better diagnosing Parkinson’s. Machine learning, at its most basic level, works by feeding a computer a large amount of data alongside a set of mathematical rules or algorithms that the computer uses to learn from the data.
Previous attempts to use machine learning to make sense of EEG have yielded promising results, but the tools have some notable limitations. One of the biggest has to do with the fact that EEG readings aren’t static. The electrical activity in the brain fluctuates over time, and previous machine learning algorithms have had limited capacity to deal with this.
TFR combined with AlexNet helps model assess data
To address this limitation, the new model uses a concept called Time-Frequency Representation (TFR), which is basically a mathematical way to represent changes in electrical activity over time in a way that can be interpreted by a computer.
The TFR setup was combined with a specific type of machine learning framework called AlexNet, named for Alex Krizhevsky, one of the computer scientists who first developed this type of machine learning algorithm. This framework was used to help make sense of readings from different electrodes, corresponding to different parts of the brain.
Combining the TFR with the AlexNet allowed this machine learning model to effectively assess data on how electrical activity in different brain parts varied over time, the researchers said.
They illustrated the utility of their model using two large datasets, showing that it was able to achieve more than 95% accuracy at distinguishing between people with or without Parkinson’s. The team noted that readings from the front and center parts of the brain were especially key in the model’s ability to identify Parkinson’s.
The model yielded better accuracy than earlier machine learning tools that didn’t include data about electrical activity over time.
“These results highlighted the superiority of the TFR-based AlexNet … model over existing methods in effectively classifying [Parkinson’s] -related EEG data,” the scientists wrote.