Machine learning aids distinguishing Parkinson’s, essential tremor voice
Researchers used machine learning to analyze speech from different languages
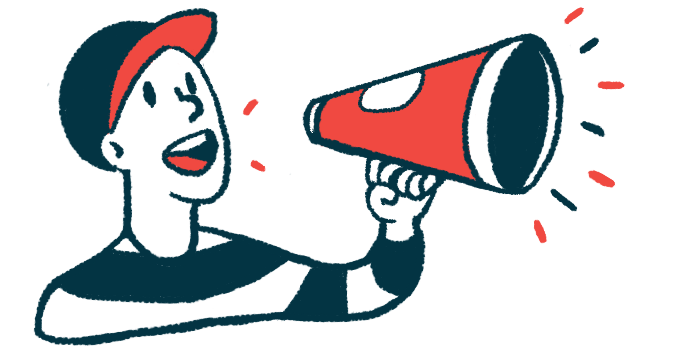
Using machine learning to analyze speech from speakers of different languages could help distinguish between Parkinson’s disease and essential tremor, two neurological disorders that can cause similar symptoms.
That’s according to the study, “Automatic speech-based assessment to discriminate Parkinson’s disease from essential tremor with a cross-language approach,” which was published in npj Digital Medicine.
Parkinson’s and essential tremor are distinct diseases with many common symptoms, most notably tremor, which can make it hard to tell them apart in clinical practice.
Both Parkinson’s and essential tremor can cause speech abnormalities, but specific patterns of speech changes are distinct. Parkinson’s frequently leads to an unusually quiet, monotonous voice, whereas essential tremor can cause abnormal variations in tone and pitch due to tremors around the vocal cords.
Studies have explored using machine learning to identify Parkinson’s based on vocal recordings. This has involved feeding a computer a large dataset alongside mathematical rules, called algorithms, which the computer uses to identify patterns in the data. The computer can then use these “learned” patterns to make sense of new sets of data.
Analyzing voice in different languages
Prior work has used only speakers of one language to train and test the computer. In this study, scientists created machine learning algorithms that could work across languages. Specifically, they trained their machine learning tool using two datasets, one of Spanish speakers and one of German speakers. They then used the tool to analyze Czech speakers.
For each patient in the analysis, two types of vocal recordings were analyzed, one where patients monologued in their native tongue and another where they uttered a series of nonsense syllables.
Analyses of the monologue recordings were generally more accurate when the computer was trained on the German-speaking database and less accurate with the Spanish-speaking database. This is likely because Czech, a Slavic language, is more linguistically similar to German than to Spanish, a romance language. In the syllable recordings where all the participants were saying the same sounds, the computer had similar accuracy with Czech speakers when trained on either Spanish or German speakers.
In initial tests that compared Parkinson’s against essential tremor, the researchers found their tool could identify patients with an accuracy of up to 81.4% for the monologue recordings and 86.2% for the syllables.
The tool was less accurate in further tests that included people with Parkinson’s or essential tremor alongside people without neurological disease. There was an overall accuracy of 63.3% and 71.6% for the monologue and syllables, respectively. The lower accuracy was largely due to a poor ability to distinguish people with Parkinson’s from healthy controls, whereas people with essential tremor were generally classified accurately.
“Our findings suggest that automated speech analysis, combined with machine learning is robust, accurate, and can be adapted to different languages to distinguish between [Parkinson’s] and [essential tremor] patients,” said the scientists, who noted more research could validate and expand their findings.