Machine-learning analysis of videos may help identify Parkinson’s
Computer-based approach may detect disease-related movement problems
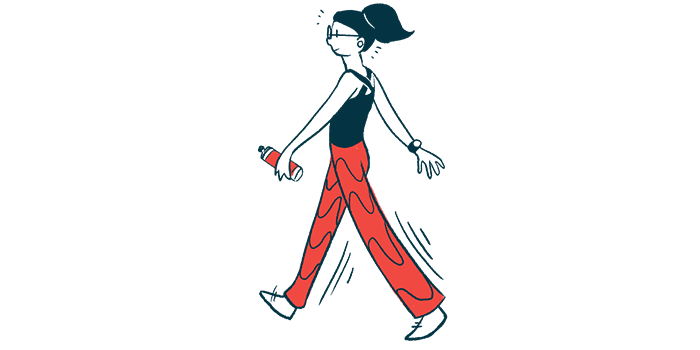
Conducting a machine-learning analysis of video recordings may aid in identifying motor symptoms in the early stage of Parkinson’s disease, potentially helping diagnose patients faster than can be done with the human eye, a new study reports.
Such computer-based analyses may be able to detect problems with walking and other physical symptoms indicative of the neurodegenerative disease early on — “by comparing the movement of the left and right sides of [the] body,” according to a press release by the researchers, from the University of Florida (UF).
“The technique is noninvasive, uses standard video recordings, and could potentially help in detecting signs of Parkinsonism earlier, improving treatment outcomes and patient management,” said Diego L. Guarín, PhD, lead author of the study and an assistant professor of applied physiology and kinesiology at the UF College of Health and Human Performance.
Indeed, the study found that machine-learning analyses combining several algorithms had accuracy as high as 86%.
The study, “What the trained eye cannot see: Quantitative kinematics and machine learning detect movement deficits in early-stage Parkinson’s disease from videos,” was published in the journal Parkinsonism and Related Disorders.
Machine learning analysis had overall accuracy over 85% in study
Parkinson’s is marked by motor symptoms such as bradykinesia, or slowed movement, as well as tremor and rigidity. The disease is diagnosed by looking for these characteristic motor symptoms — which means the diagnosis can be a bit subjective and usually relies on a highly trained expert. There aren’t many such experts, which can be a major obstacle for people trying to confirm a diagnosis.
A potential solution to this problem would be to use computer-based analyses of videos, thereby minimizing the need for a human expert. This would also allow for videos to be made when potential signs of the disease are more evident, as symptoms oftentimes don’t present themselves in a doctor’s office or clinic.
Now, a team of UF researchers explored this approach using machine learning, which is a technique in which computers are fed large amounts of data alongside mathematical rules that the computer uses to learn from the information provided, identifying patterns that can then be used to make sense of new data.
The team noted that using machine learning to assess motor symptoms “provides an accessible, simple, and cost-effective approach that complements traditional clinical care.”
[These findings] provide evidence supporting the idea that using [machine learning] algorithms for video-based movement estimation is a viable approach for detecting motor alterations corresponding to early-stage [Parkinson’s] from videos.
For this study, video recordings from 31 people with early-stage Parkinson’s and 26 people of similar ages but who did not have Parkinson’s were analyzed. All of the participants had a mean age in the early 60s.
The researchers specifically focused on people with early Parkinson’s — those, on average, fewer than three years out from diagnosis — noting that other studies of machine learning have generally involved Parkinson’s patients at various stages. Using patients across disease stages makes the data messier, the team noted, as Parkinson’s symptoms can change a lot over time.
Videos were taken of patients doing three tasks — finger tapping, hand movement, and leg agility — that are part of the Unified Parkinson’s Disease Rating Scale (UPDRS), a standardized clinical assessment used to measure the severity of Parkinson’s symptoms. With UPDRS, symptom severity usually is judged by an expert.
The scientists specifically focused on videos of participants’ hands and legs based on left-side and right-side movements as well as right and left symmetry. This is because Parkinson’s tends to affect patients asymmetrically, meaning one side of the body is impacted more than the other.
By integrating left side, right side, and symmetry features, the machine learning algorithms were able to distinguish Parkinson’s patients with an accuracy of 79% using finger tap videos, 75% using hand movement videos, and 79% using leg agility videos. When combining all three tasks, the detection accuracy increased to 86%. Overall, 97% of Parkinson’s patients and 73% of those without the disease were correctly classified, the team noted.
These findings “provide evidence supporting the idea that using [machine learning] algorithms for video-based movement estimation is a viable approach for detecting motor alterations corresponding to early-stage [Parkinson’s] from videos,” the researchers wrote.
“Such an approach might provide a much-needed support to providers (including primary care physicians or general neurologists) to screen and evaluate patients with early or mild signs of motor dysfunction when parkinsonism is suspected,” the team added, noting that there is “a critical shortage of sub-specialist with expertise in [Parkinson’s].”