EEG patterns put to AI review may aid in early Parkinson’s diagnosis
Proposed model shows good accuracy, specificity in tests
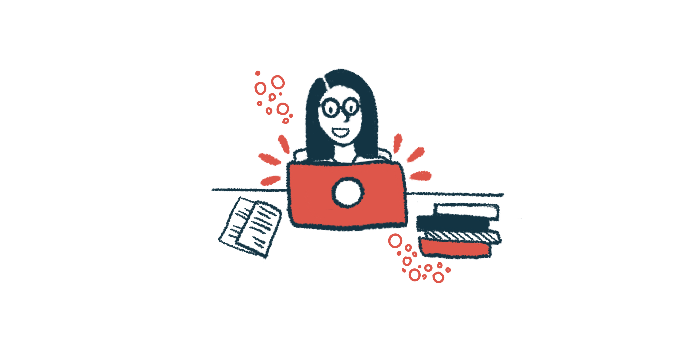
A model that uses artificial intelligence (AI) to analyze electroencephalography (EEG)-based patterns of brain activity showed a potential to accurately diagnose Parkinson’s disease at early stages, according to a recent study from Australia.
EEG, a non-invasive way of studying the brain’s electrical activity, is used to detect patterns of activity that could be associated with neurological diseases.
“By developing advanced AI-based techniques, we aim to enhance early detection and ultimately improve the management of this devastating condition,” Siuly Siuly, PhD, a research fellow at Victoria University in Melbourne and the study’s first author, said in a university press release.
Study findings will help in creating “an essential technology for efficient PD [Parkinson’s disease] diagnosis, enhancing patient care and quality of life,” the researchers wrote in “An efficient Parkinson’s disease detection framework: Leveraging time-frequency representation and AlexNet convolutional neural network,” published in Computers in Biology and Medicine.
Parkinson’s often diagnosed when symptoms like rigidity are evident
Parkinson’s disease is caused by the progressive dysfunction and death of dopaminergic neurons, nerve cells responsible for producing the neurotransmitter dopamine. Dopamine plays a vital role in facilitating communication between neurons and is essential for proper motor control.
The diagnosis of Parkinson’s primarily is based on the presence of motor symptoms like bradykinesia (slowed movement), muscle rigidity, resting tremors, and balance and posture difficulties. However, these symptoms often are most apparent in later disease stages, meaning a start to Parkinson’s treatment often follows some degree of progression.
As Parkinson’s “persists as a lifelong condition, early detection, prompt diagnosis, and appropriate treatment are vital in enabling affected individuals to lead fulfilling lives,” the scientists wrote.
Neurological examinations and brain imaging techniques, including EEG, can be used to support a Parkinson’s diagnosis or rule out other conditions. EEG is of particular importance, the researchers noted, as abnormalities in brain wave patterns can allow researchers to further explore the disease’s underlying mechanisms, and help to track progression.
Researchers developed and trained an AI model to analyze EEG data with the goal of arriving at more accurate and efficient Parkinson’s diagnoses.
They used time-frequency representation — a view of a signal represented over both time and frequency — based on the AlexNet convolutional neural network (CNN). “AlexNet CNN is utilized to detect complex spatial patterns at different scales, accurately identifying intricate EEG patterns associated” with Parkinson’s, the scientists wrote.
Researchers also used a specific type of AI that’s designed to process and analyze data that has a grid-like structure, such as images or time-frequency representations, enabling scientists to identify and classify distinct EEG patterns linked to Parkinson’s disease.
Potential seen in artificial intelligence to ‘revolutionize’ healthcare
“Our interest in using AI as a diagnostic tool stems from the recognition of its potential to revolutionize healthcare by providing faster, more accurate, and cost-effective diagnoses,” Siuly said. “AI has shown remarkable capabilities in analyzing complex datasets like … EEG data quickly, automatically, and accurately.”
The team developed a computational model for Parkinson’s using EEG signals. They aimed to detect the disease using an efficient and straightforward model, localize the brain regions responsible for representative EEG signals for Parkinson’s detection, and enhance its performance to overcome the limits of currently available methods.
Scientists tested their model using datasets collected at two sites: one from the University of Iowa with EEG records covering 14 healthy individuals and 14 Parkinson’s patients, and another from the University of San Diego that included data on 16 healthy adults and 15 Parkinson’s patients.
Analysis of both datasets revealed that EEG patterns derived from the frontal and central brain regions were more effective in providing distinguishing features for a Parkinson’s diagnosis than other brain regions.
The proposed model achieved a high accuracy, sensitivity, specificity, and positive predictive value for both data sets. Sensitivity refers to the ability of a test to correctly identify patients, while specificity is the ability of a test to correctly identify people without the disease. Positive predictive value represents the proportion of individuals with a positive test result who are accurately diagnosed with the disease.
Specifically, this newly developed method was able to detect Parkinson’s disease with an accuracy of 99.8% in the San Diego dataset and 95.8% in the Iowa dataset, outperforming existing EEG-based detection methods for the disease’s diagnosis.
Based on these results, researchers aim to collaborate with healthcare and software professionals to develop a specialized software for clinical use. Their ultimate goal is to create a tool that aids in diagnosing Parkinson’s and that might be adapted to detect and diagnose other neurological conditions.
“This is a dynamic area of research and has the potential to revolutionize how we detect and treat significant health challenges,” Siuly said.