Analyzing Finger Taps, Voice on Cellphone May Help in Diagnosis
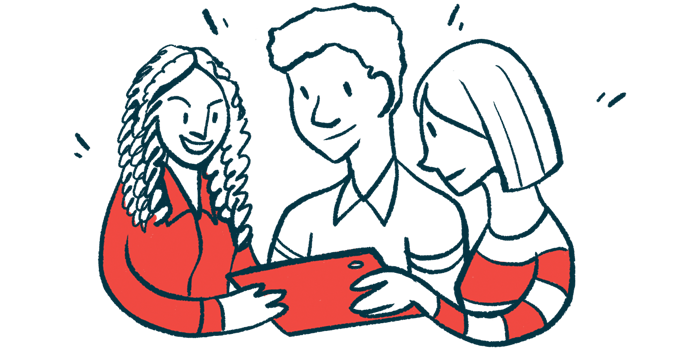
Collecting finger tapping, voice, and walking data on a smartphone to be digitally processed by deep machine learning tools can accurately distinguish between people with and without Parkinson’s disease, a study of more than 7,000 people reported.
This approach, known integrative digital biomarkers, may help to diagnose the disease in its early stages and provide a more convenient way to monitor patient symptoms and progression, its scientists suggested.
The study, “Heterogeneous digital biomarker integration out-performs patient self-reports in predicting Parkinson’s disease,” was published in the journal Nature Communication Biology.
Tremors, slow movements, and rigidity are hallmark symptoms of Parkinson’s disease.
Mobile devices and built-in sensors can convert these abnormal movements into digital signals to measure and monitor symptoms.
But studies using digital biomarkers have mainly focused on gross motor skills, such as walking and rest. People with Parkinson’s experience difficulties in fine-motor skills, including picking up objects, tying shoelaces, and writing. Poor fine-motor coordination is often a more sensitive indicator of Parkinson’s than changes in gait or balance, especially in early disease stages.
Researchers based at the University of Michigan report developing a digital biomarker system that uses machine learning computer algorithms to assess gross and fine motor skills and changes in voice that can distinguish between those with and without Parkinson’s.
The team used data from mPower, a two-year study in adults in the U.S. using a smartphone app designed to collect walking, voice, and finger tapping data from individuals with and without Parkinson’s.
Tapping data was collected — a total of 78,879 records — from 1,060 people self-reporting a confirmed Parkinson’s diagnosis and 6,418 self-reported healthy individuals. During the tapping task, participants alternately tap two fingers of their dominant hand within two squares on the touchscreen of a phone, placed flat on a table, as quickly as possible for 20 seconds.
Predictive models were then calculated from accelerometer data.
“When participants tap on the screen, the tapping motion induces acceleration of the phone,” the researchers wrote. “Thus, accelerometer data is capable of capturing the magnitude, direction and speed for the movement of the phone.”
After applying machine learning, the ability of the accelerometer data to predict Parkinson’s reached what’s called a mean area-under-the-curve (AUC) value of 0.9174. Of note, AUC is a method to assess a test’s accuracy, in which an AUC of 0.7 to 0.8 is considered acceptable, 0.8 to 0.9 is good, and more than 0.9 is deemed excellent.
Next, mPower was used for what is called coordinate data, collecting the location of movements during those taps on the cellphone. Here, Parkinson’s patients were expected to exhibit poorer coordination as well as slower motion than healthy individuals. After applying machine learning, the ability of the tapping coordinate model to predict Parkinson’s reached a mean AUC of 0.9352.
Researchers then compared the tapping models with gait/rest and voice models for predicting Parkinson’s. A total of 2,729 participants had all three types of data, including 645 people with Parkinson’s.
Cellphone accelerometer movement data during 20- or 30-second walking and resting (standing still) activities were analyzed using the deep learning network. Walking data reached an average AUC of 0.8983. mPower voice data isolated from recordings of the participants saying ‘Ahh’ for 10 seconds achieved an average AUC of 0.8335.
Using data from this smaller patient group only, the accelerometer deep learning model led to an average AUC of 0.8983, while the tapping model generated an average AUC of 0.9236. Combining accelerometer and coordinate tapping models achieved a better performance than either model alone, with an average AUC of 0.9333.
“We found that tapping models significantly outperformed voice and gait/rest models in this population,” the researchers wrote.
Combining data across all four models (accelerometer tapping, coordinate tapping, walking, and voice) led to an AUC of 0.944, which was better than each individual model, suggesting that “the evaluation scores of different models may have specific limitations and that assembling all sources of information may … obtain better performance,” they added.
The performance was similar among different sexes and smoking groups, except for those 35 years old or younger. All machine learning model AUCs for participants older than 35 were greater than 0.85, and 0.885 for those older than 45. Overall, the prediction values increased with age due to more Parkinson’s patients included in the sample, the scientists noted.
Lastly, the team compared their prediction models against the Unified Parkinson’s Disease Rating Scale (UPDRS), again based on patient self-reports. Among those with available data, the machine learning model reached an AUC of 0.9486, whereas the AUC based on UPDRS was 0.8232.
When UPDRS Part 2 scores were used, which assesses motor function specifically, the AUC was 0.9356, suggesting that “digital biomarkers can make more accurate predictions than patient self-reports,” the researchers concluded.
In addition, machine learning prediction strongly correlated with both UPDRS scores and with UPDRS Part 2 scores, supporting the clinical relevance of the deep learning model, they added.
“Our results indicate an integrative digital biomarker approach with several potential applications for [Parkinson’s disease] detection and monitoring of disease activities,” the scientists wrote. “Our study also emphasizes the advantages of utilizing different types of biomarkers.”
Future work could “include a collection of long-term follow-up data to evaluate the model ability of predicting [Parkinson’s disease] before diagnosis, and a collection of other movement disorders,” the team noted.
The study was funded in part by Eli Lilly, along with the American Parkinson’s Disease Association, the Michael J. Fox Foundation, and the Parkinson’s Foundation. The mPower study was developed by Sage Bionetworks.