Contest leads to 5 new models of wearable sensors for freezing of gait
Winners of global machine-learning contest in Parkinson's split $100K prize
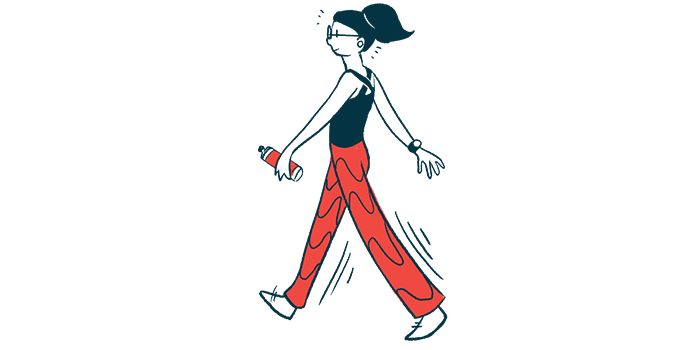
Five top models for a wearable sensor that can monitor and measure freezing of gait (FOG) — a sudden inability to walk — in people with Parkinson’s disease were developed as the result of a three-month, international machine-learning contest launched by scientists at Tel Aviv University in Israel.
The teams behind these winning models, which the scientists say potentially “could replace or augment visual, time-consuming video [analysis] by expert reviewers” — the current gold standard for FOG assessment — split a $100,000 prize.
“This successful endeavor underscores the potential of machine learning contests to rapidly engage AI [artificial intelligence] experts in addressing critical medical challenges and provides a promising means for objective FOG quantification,” the scientists wrote, adding that “the winning efforts markedly improved previous [FOG] detection abilities.”
According to Jeffrey M. Hausdorff, PhD, the study lead at the university’s faculty of medical & health sciences and the Sagol School of Neuroscience, the models were based on machine learning, in which computer programs learn from inputted data.
“Wearable sensors supported by machine learning models can continuously monitor and quantify FOG episodes, as well as the patient’s general functioning in daily life. This gives the clinician an accurate picture of the patient’s condition at all times,” Hausdorff said in a university press release, noting using such devices would allow healthcare providers to see if patients respond to prescribed medications.
“The informed clinician can respond promptly, while data collected through this technology can support the development of new treatments,” Hausdorff said.
The contest and its outcomes were detailed in a study titled “A machine learning contest enhances automated freezing of gait detection and reveals time-of-day effects,” published in Nature Communications.
FOG detection challenge launched for machine learning community
Gait refers to a person’s manner of walking. Freezing of gait, or an inability to lift the foot and take a step — known as FOG for short — is a disabling symptom that affects up to 65% of people with Parkinson’s.
“A FOG episode can last from a few seconds to more than a minute, during which the patient’s feet are suddenly ‘glued’ to the floor, and the person is unable to begin or continue walking,” said Hausdorff, who’s also at the Center for the Study of Movement, Cognition and Mobility at the Tel Aviv Medical Center.
“FOG can seriously impair the mobility, independence, and quality of life of people with Parkinson’s disease, causing great frustration, and frequently leading to falls and injuries,” Hausdorff noted.
The gold standard for assessing FOG, used in addition to self-report questionnaires and visual observation by clinicians, is a frame-by-frame video analysis of patients showing them moving. While accurate, such video assessment has limitations, such as the need for multiple specialists. It’s also time-consuming. Moreover, long-term video monitoring inside the home is impractical.
Although there is a growing effort to develop wearable sensors to track and measure a patient’s daily functioning, “successful trials have all relied on a very small number of subjects,” Hausdorff said.
Such constraints led Hausdorff and colleagues to launch a FOG detection challenge for the machine learning community. The goal was to accelerate the development of a reliable, cost-effective, and automatic detection method for freezing of gait.
A prize of $100,000, divided among the top five finishers, was funded by the Michael J. Fox Foundation for Parkinson’s Research and Kaggle, a Google company that conducts international machine learning competitions. Altogether, there were 24,862 submissions from 1,379 teams across 83 countries.
The participants created machine-learning models based on data collected from Parkinson’s patients wearing a single lower-back sensor and from video analysis, which recorded about 5,000 FOG episodes in total. The contest’s parameters were to detect and classify three types of freezing of gate events: start hesitation, FOG during turns, and FOG episodes during walking.
Freezing of gait most likely to occur at about 7 a.m. and 10 p.m.
Submissions were ranked based on performance against two data sets with 40 Parkinson’s patients and more than 1,300 validated FOG episodes. All five of the top models showed good accuracy in detecting all FOG classes, ranging from 88% to 92%.
The best performance was for FOG during turns, the most common FOG class. Still, there was a trade-off across the models between successfully detecting walking events and start hesitation. Freezing of gait episodes during walking were the hardest to detect, while start hesitation performance was generally better. Ultimately, the top model best balanced these two classes.
A correlation analysis then compared the model estimates with the actual percent time frozen (%TF), the number of freezing episodes, and the duration of each episode per patient, as assessed by video.
All five models show good to excellent statistically significant %TF correlations, “indicating that the models accurately estimated the percentage of the total time spent freezing,” the researchers wrote. The correlation for the total FOG duration also was excellent, but was weaker for the number of freezing episodes.
The contest we initiated brought together capable, dynamic teams all over the world, who enjoyed a friendly atmosphere of learning and competition for a good cause. … Rapid improvement was gained in the effective and precise quantification of FOG data.
Finally, the team estimated the %TF over time between Parkinson’s patients with and without FOG, as assessed during a clinical visit or the patient-reported new freezing of gait questionnaire.
In the FOG group, there was a significant difference between the hourly %TF during the daytime and the median %TF at night. Moreover, there appeared to be two peaks of freezing episodes — at about 7 a.m. and 10 p.m.
“We observed, for the first time, a recurring daily pattern, with peaks of FOG episodes at certain hours of the day, that may be associated with clinical phenomena such as fatigue, or effects of medications,” said Eran Gazit, a co-author of the study from the Tel Aviv Medical Center. “These findings are significant for both clinical treatment and continued research about FOG.”
Hausdorff noted that “rapid improvement was gained in the effective and precise quantification of FOG data.”
But for this scientist, there was another very important result: “Our study demonstrates the power of machine learning contests in advancing medical research,” Hausdorff said.
“The contest we initiated brought together capable, dynamic teams all over the world, who enjoyed a friendly atmosphere of learning and competition for a good cause, Hausdorff said. “Moreover, the study laid the foundations for the next stage: long-term 24/7 FOG monitoring in the patient’s home and real-world environment.”