Blood Metabolites May Help Diagnose Men With LRRK2 Mutations
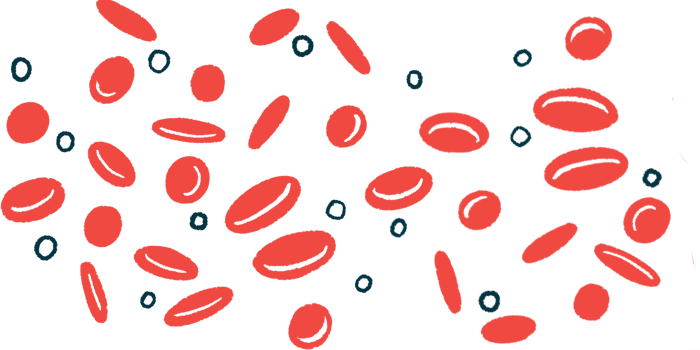
Metabolomics — global analyses of the many small, biologically relevant molecules in blood — may help to identify males with Parkinson’s disease who carry a mutation in the LRRK2 gene, allowing for earlier diagnosis, a study reported.
The study, “Plasma Metabolite Signature Classifies Male LRRK2 Parkinson’s Disease Patients,” was published in Metabolites. It was led by scientists at the company Olaris.
Blood, other bodily fluids, and tissues are abundant in small molecules called metabolites (e.g., small sugars or pieces of proteins) that are produced and used in various bodily processes. Global analyses of these metabolites, called metabolomics, has gained increasing interest in recent years as a method for analyzing health and disease.
“Metabolomics is the comprehensive analysis of low‐molecular‐weight molecules that provide energy and biomass for life to exist,” the researchers wrote.
Some studies have suggested differences in metabolites among Parkinson’s patients, and that metabolomic analyses could be helpful for diagnosing the disease. However, this type of research is still largely in its infancy.
Scientists collected blood samples from 46 Parkinson’s patients and, for comparison, 65 people without Parkinson’s of similar age and sex as controls. Notably, all the patients had a Parkinson’s-causing mutation in the LRRK2 gene called G2019S. Mutations in this gene, which provides instructions for making an enzyme of the same name, account for about 5% of familial Parkinson’s cases, and about 1% of sporadic cases.
Sophisticated chemical analyses of the samples were conducted to look at global changes in various metabolites. Other analyses looked for differences between patients and controls.
Most study participants were male, which was expected as Parkinson’s is more common in men. Since a person’s biological sex can influence their metabolomic profile, analyses were conducted both with males and females together and separately by sex.
The most noteworthy findings were identified in the analysis that only looked at males, which included 32 men with Parkinson’s and 42 controls. (Findings in women might have been affected by these groups’ small size, 13 female patients and 22 controls, the study noted.)
Significant differences were evident between the male patients and controls for 18 metabolic resonances. The team noted that a “resonance” is not an exact measure of a metabolite’s level due to certain technical considerations, but “the intensity for each resonance can be used as a proxy for relative concentration.”
Of these 18 resonances, 11 were higher in Parkinson’s patients than in controls, while the other seven were lower in patients than controls.
Closer analyses suggested that many of the metabolites that were dysregulated in patients were amino acids — small molecules that are strung together to form proteins — while others related to making or generating energy from glucose (a form of simple sugar).
“Many of these metabolites have been previously identified as altered in PD [Parkinson’s disease], suggesting that flux through these pathways could be an important contributor to PD biology,” the scientists wrote.
Researchers then applied machine learning to the metabolic data to generate diagnostic algorithms. This basically involves giving a computer about two-thirds of the data, along with some mathematical rules so the computer can “learn” ways to sort Parkinson’s from non-Parkinson’s samples.
The remaining data was used to subsequently test these rules with a statistical measure called the AUC (area under the receiver operating characteristic curve). AUC values can range from 0.5 to 1, with higher values indicating a better ability to differentiate between two groups — here, Parkinson’s or not.
After testing several different mathematical models and making some refinements, the researchers’ algorithm had an AUC of 0.83. Of note, the algorithm failed when applied to data covering female patients and controls.
“While there were a few [male] patients and controls who fell outside the score cutoff for non‐PD vs. PD status, the majority were correctly predicted using the score,” the researchers concluded. They noted that further testing and gathering more data, as well as expanding to other sexes and subtypes of Parkinson’s, could help to refine the algorithm.