AI model may predict brain age in Parkinson’s by stiffness, volume
Data was collected from 279 people to detect brain properties
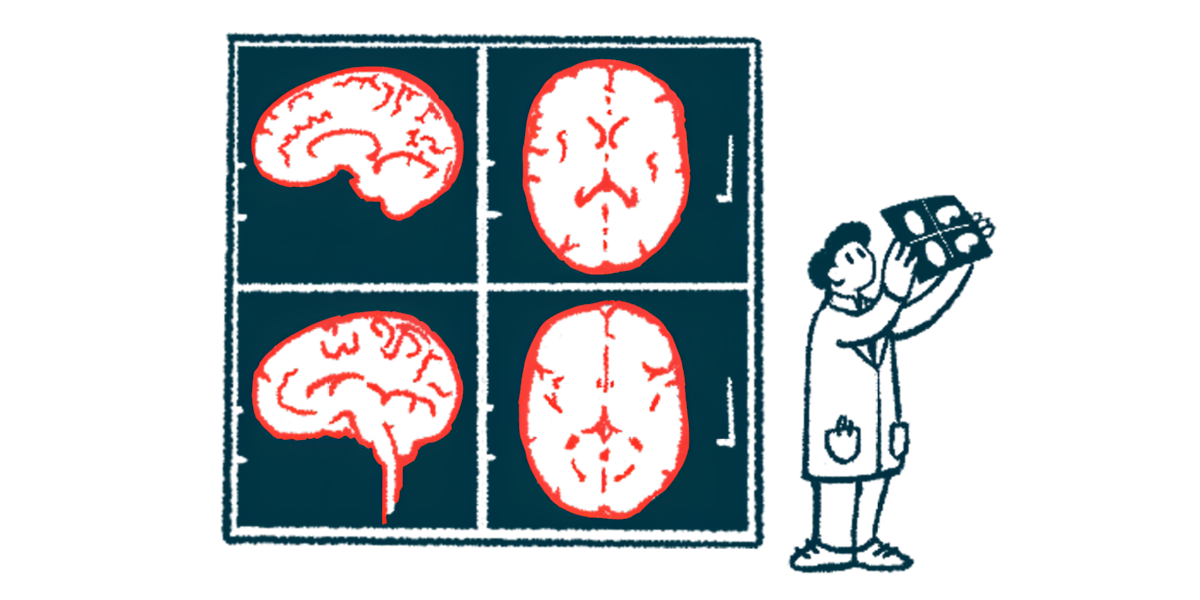
Researchers at the University of Delaware have developed a model that combines artificial intelligence with magnetic resonance elastography (MRE) to measure brain stiffness and volume for predicting brain age.
MRE is a noninvasive method that can measure changes in brain stiffness by using a gentle vibration of a person’s head while they’re being scanned using MRI. This helps create a brain stiffness map based on how tissue in each part of it responds to a light touch.
As the brain tends to be less stiff and grow softer as people age or have neurodegenerative disorders such as Parkinson’s disease, this model could help detect structural changes that indicate deviations from normal aging.
“The results demonstrate the complementary value of MRE measurements in brain age models, which, in future studies, could improve model sensitivity to brain integrity differences in individuals with neuropathology [nervous system disease],” the researchers wrote. The findings were described in “MRI-based whole-brain elastography and volumetric measurements to predict brain age,” in Biology Methods & Protocols. In the study, the researchers showed that measuring both brain stiffness and volume resulted in accurate predictions of a person’s age.
“Brain volume is a common measure that we use to study the brain,” Curtis Johnson, PhD, professor of biomedical engineering at University of Delaware and co-senior author of the study, said in a university news story. “But something has to be happening to cause a brain to shrink. Something is happening at the microscale that causes it to shrink — changes in the tissue that also cause stiffness to change. And that precedes whatever happens when the volume changes.”
Creating a ‘matrix of stiffness’
The researchers collected data from 279 participants with a mean chronological age, that is, age as measured from birth, of 31, using MRE to detect brain mechanical properties, including stiffness, and high-resolution scans to assess brain volume.
“The stiffness maps all seem kind of random — until we see a large number of images and the randomness fades away and we start to see common patterns in stiffness,” Johnson said. “We sort of knew there was more [information] in there than what we were extracting. But the traditional way of interacting with the stiffness data is pretty crude.”
The scientists used three-dimensional convolutional neural networks to detect changes in brain stiffness and compared their performance to that of more traditional statistical models.
In data analysis and neuroscience, neural, or nerve cell, networks help recognize patterns and establish predictive models, using artificial intelligence.
“The neural network in your cortex — all of those little neurons connecting — that’s creating this matrix of stiffness in your brain,” said Austin Brockmeier, PhD, professor of electrical and computer engineering at the University of Delaware and co-senior author. “Every time you learn something new and your brain rewires, that is what’s creating this stiffness. When many neurons are connected between brain areas they get stiff like a cable.”
The investigators found that combining measurements of brain stiffness and volume yielded the most accurate chronological age predictions than using volume alone.
“While volume reflects macroscale structural changes, stiffness offers insights into microstructural health, and together they provide a more comprehensive picture of brain aging,” the researchers wrote. “This highlights the importance of leveraging multiple brain imaging modalities to improve the accuracy of age prediction models.”