AI Analyzes Facial Expressions in Videos to Help Detect Parkinson’s
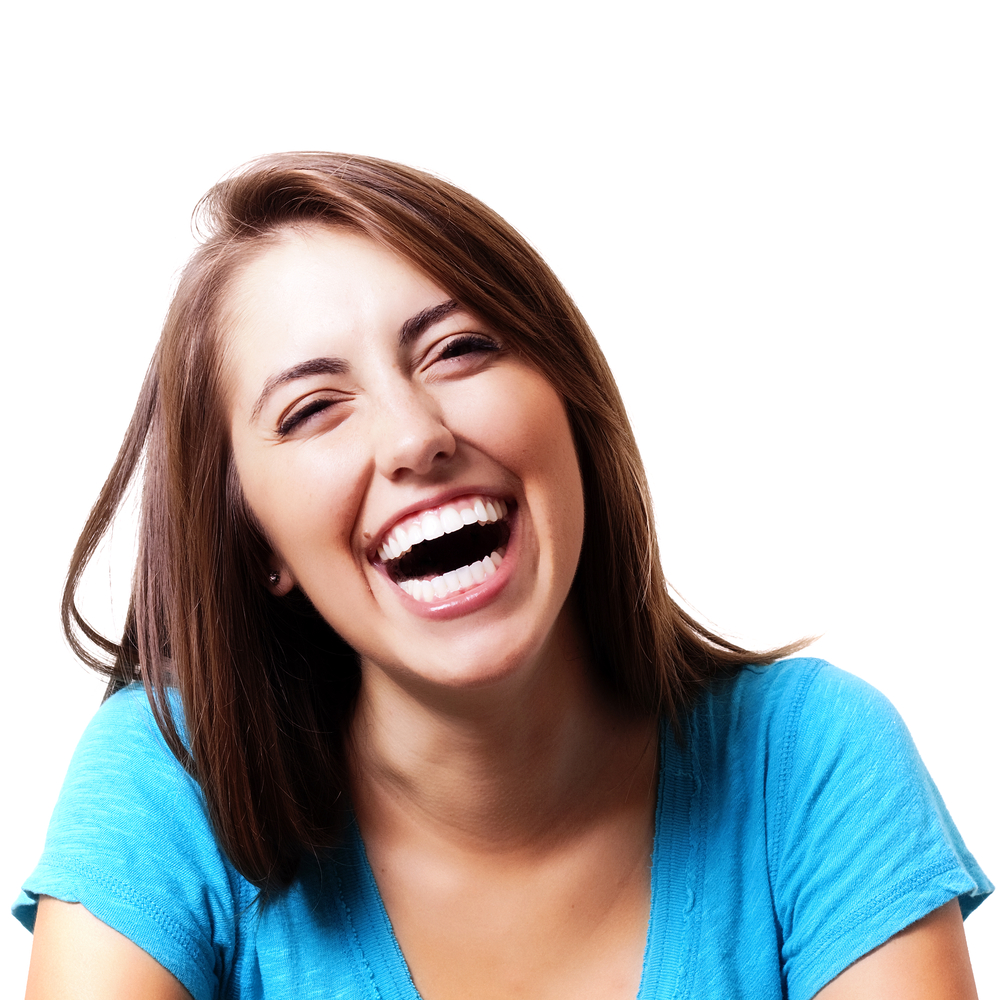
Dayna More/Shutterstock
An artificial intelligence (AI) tool was able to distinguish, with great accuracy, Parkinson’s patients from healthy peers by analyzing short videos of facial expressions, particularly smiles, a small study shows.
The predictive accuracy of the new tool was comparable to that of video analysis that uses motor tasks to detect Parkinson’s, pinpointing facial expressions as a potential digital, diagnostic biomarker of the disease.
This type of biomarker could allow remote diagnosis without the need for personal interaction and extensive testing. This would be particularly relevant in situations such as a pandemic, in cases of reduced mobility, or in underdeveloped countries where few neurologists exist but most people have access to a phone with a camera, researchers noted.
The study, “Facial expressions can detect Parkinson’s disease: preliminary evidence from videos collected online,” was published as a brief communication in the journal npj Digital Medicine.
Parkinson’s-associated motor symptoms, such as tremors and muscular rigidity, affect facial muscle movements, leading to overall reduced facial expression, also known as facial masking or hypomimia.
An increasing number of studies suggest that reduced facial expressions may be an “extremely sensitive biomarker for [Parkinson’s disease], making it a promising tool for early diagnosis,” the researchers wrote.
In addition, facial expression analysis is a non-invasive tool that only requires a webcam or a phone with a camera, in contrast to the expensive, non-scalable, wearable sensors currently used to analyze movements during motor tasks as digital biomarkers of Parkinson’s.
Now, a team of researchers at the University of Rochester, New York, showed that analyses of facial micro-expressions using an AI tool can accurately detect Parkinson’s.
The study involved the analysis of 1,812 videos, collected online through a web-based tool (Park test), of 604 people (61 with Parkinson’s and 543 without the disease). In these videos, participants were asked to make three facial expressions — a smiling, disgusted, and surprised face — each followed by a neutral face.
Participants’ mean age was 63.9 years, and most of them were white and living in the U.S. Patients with Parkinson’s had been living with a diagnosis of the disease for a mean of 8.4 years.
Changes in muscle movements in each of the three facial expressions were objectively measured and computed in terms of nine action units, or micro-expressions.
In agreement with previous research, the analysis showed that Parkinson’s patients had fewer facial muscle movements than people without the disease. This was particularly significant for three micro-expressions: raising cheeks and pulling the lip corner — typically observed when people smile — and lowering the brows, usually seen when people express a disgusted face.
According to the team, “the smiling facial expression has the greatest potential in differentiating individuals with and without” Parkinson’s, the researchers wrote.
The team then used these differences in micro-expressions to train a machine learning tool to distinguish between individuals with and without Parkinson’s. Machine learning is a form of AI that uses algorithms to analyze data, learn from its analyses, and then make a prediction about something.
They found that their AI tool could correctly identify Parkinson’s patients based on their facial expressions with an accuracy of 95.6%, which is comparable to the 92% prediction accuracy reported for existing state-of-the-art video analysis that relies on limb movements.
“We show that an algorithm’s ability to analyze the subtle characteristics of facial expressions, often invisible to a naked eye, adds significant new information to a neurologist,” the team wrote.
As such, facial expressions, especially smiling, “may become a reliable biomarker for [Parkinson’s disease] detection,” they added.
This type of diagnostic biomarker does not require in-person examination and diagnosis, which may be “potentially transformative for patients need of remote diagnoses due to physical separation (e.g., due to COVID) or immobility,” the team wrote.
In addition, “many underdeveloped areas and underrepresented populations can also benefit from such method that utilizes facial expressions without worrying about direct access to a neurologist,” the researchers added.
They noted that in 23 African countries, the mean population per neurologist exceeds half a million, but about 75% of people in Africa have access to a mobile phone, and that similar situations exist in Asia and South America.
Moreover, the authors hypothesized the potential value of an app, that, with the user’s permission, could automatically analyze the micro-expressions of the user’s smile (from a short video) and provide a referral in case there were signs of Parkinson’s risk.