Way of Detecting Parkinson’s Early via Typing Patterns Being Tested and Refined
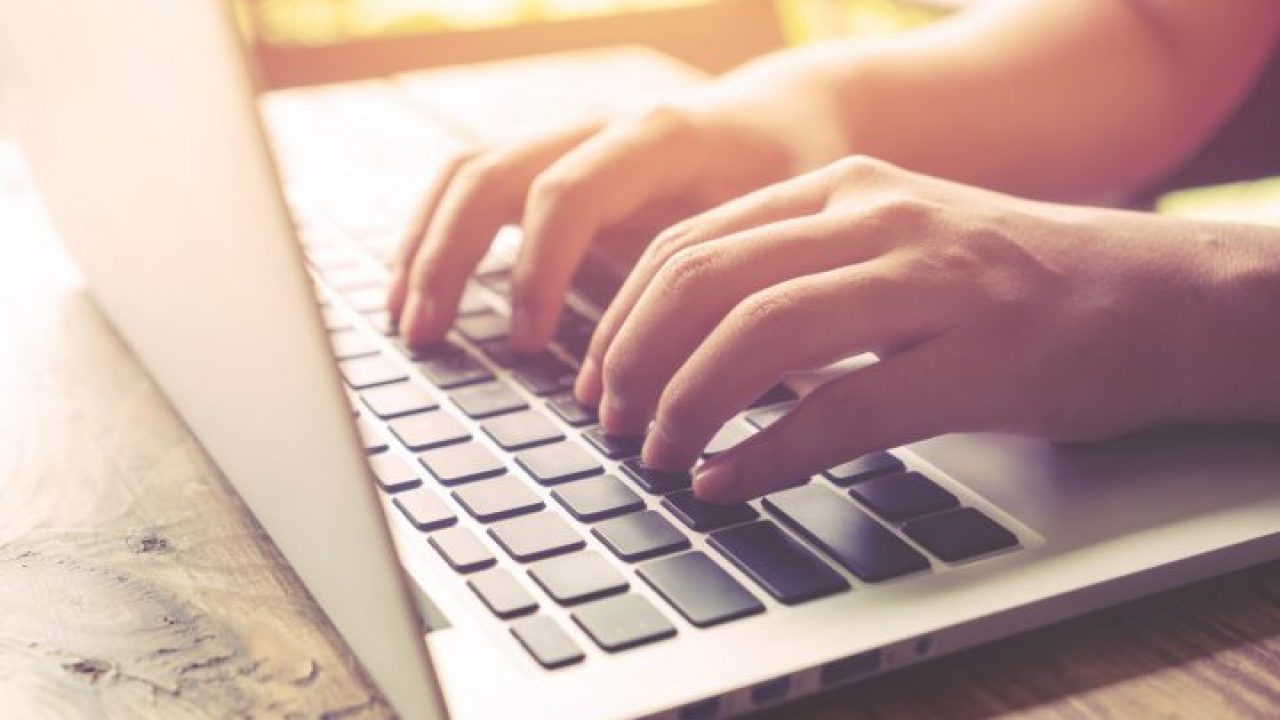
A type of computational analysis that works to analyze typing patterns may help in detecting motor signs of Parkinson’s disease at early stages, the researchers who developed the analysis report.
This new method, which appeared to allow researchers to discriminate between people with early Parkinson’s and those without the disease, may also speed data collection and analysis of disease states across neurodegenerative ills.
The study, “Classification of Short Time Series in Early Parkinson’s Disease With Deep Learning of Fuzzy Recurrence Plots,” was published in the IEEE/CAA Journal of Automatica Sinica.
Objective measures of Parkinson’s motor signs are crucial for diagnosing the disease early and correctly, as well as for monitoring progression and assessing treatment response. Early detection of Parkinson’s disease (PD) is particularly relevant, as people at early stages of the disease are more likely to benefit from neuroprotective treatments.
“Because a significant amount of the [midbrain’s] substantia nigra neurons have already been lost or impaired before the onset of motor features, people with PD may first start experiencing symptoms later in the course of the disease,” the researchers wrote.
Current methods to evaluate motor symptoms focus on a person’s movements and balance while walking, which requires a trained specialist and clinic visits. As such, they limit the frequency at which disease state and progression is likely to be assessed.
In addition, these methods involve the collection of data “during relatively long walking periods, causing discomfort to the participants or impracticability of performance in clinical settings,” the researchers wrote.
Increasing efforts are being made to develop easier and more accessible methods of detecting Parkinson’s motor signs, including the use of digital technologies.
A previous study showed that analyzing the time a person takes between pressing and releasing a key while typing (key hold time) could be used to detect motor problems in the early stages of Parkinson’s. The analysis involves a computational self-learning algorithm able to generate a Parkinson’s disease motor index based on key hold times.
This approach — which measures key hold times during the normal use of a computer without any change in hardware — was shown to efficiently distinguish people with and without Parkinson’s using data from either a controlled clinic setting or an uncontrolled at-home setting.
While it has the potential to be an objective and user-convenient tool to detect Parkinson’s, this approach “require the time series of length to be considerably long.”
Researchers at Linköping University (LiU), in Sweden, developed a new way of analyzing typing patterns based on a very short time series of data for machine learning (artificial neural networks that learn from data). It intends to avoid discomfort to participants in performing long physical tasks for data recording, and to effectively differentiate Parkinson’s patients from healthy people.
The team used the first short segments of the key hold time data from 43 healthy individuals and 42 Parkinson’s patients (average time since diagnosis, 3.9 years), part of a publicly available database. Of note, patients were on parkinsonian medication, but stopped their treatments for at least the 18 hours before the typing test.
Researchers first translated the data into a set of two-dimensional, grey-scale images of texture, called fuzzy recurrence plots, which were then used for machine learning with an algorithm named long short-term memory (LSTM)-based deep learning.
The use of fuzzy recurrence plots in machine learning allowed for distinguishing among people with and without Parkinson’s using less data than current methods.
According to a press release, researchers believe their findings are “encouraging,” and plan to further explore the use of fuzzy recurrence plots and improve the algorithm to better determine a patient’s disease state.
They also highlighted that this approach may be applied to other types of data, with a goal of improving machine learning and reducing the amount of data required to achieve good results for differentiating healthy people from those with disease.
The research team plans to evaluate this approach against walking and balance data collected from people with Parkinson’s and other neurodegenerative diseases, such as Huntington’s disease and amyotrophic lateral sclerosis (ALS).