System uses sensors, machine learning to detect Parkinson’s
Simplified setup includes one sensor on the back, pared down algorithms
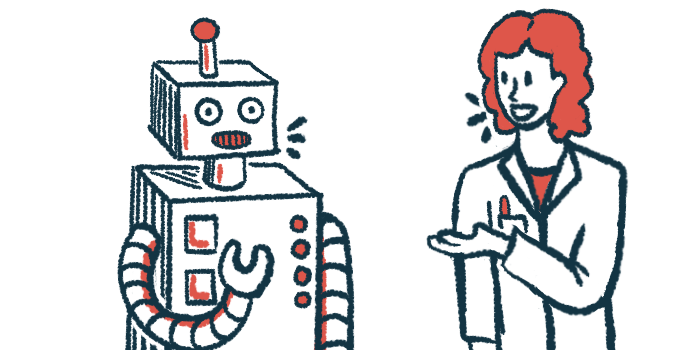
Integrating wearable sensors and machine learning has enabled researchers to develop a simplified method to diagnose Parkinson’s disease.
The technology was described in the study, “Simplification of Mobility Tests and Data Processing to Increase Applicability of Wearable Sensors as Diagnostic Tools for Parkinson’s Disease,” published in Sensors.
Diagnosing Parkinson’s is complicated. Currently, it relies on a detailed clinical evaluation where an expert assesses whether a person is showing symptoms of Parkinson’s.
To help improve the speed and objectivity of diagnoses, recent studies have explored the use of wearable sensors and machine learning. Machine learning works by feeding data into a computer alongside algorithms that the computer uses to identify patterns. The sensors collect information about patients’ movements and then machine learning is applied to detect changes typical of Parkinson’s.
Although many studies have shown that sensors and machine learning can be applied to help detect Parkinson’s, it’s often difficult to use the strategy in clinical practice because studies often rely on many specialized sensors and machine learning algorithms that require a lot of computational power. Plus, patients must typically undergo a long and rigorous battery of movement tests to get enough data for accurate predictions.
Diagnosing Parkinson’s from single mobility task
Here, researchers sought to create a simplified process for detecting Parkinson’s using wearable sensors and machine learning. The setup includes just one sensor placed on the lower back and the machine learning algorithms are pared down to the bare necessities in order to minimize computational strain.
To test the process, the researchers collected data from 262 people with Parkinson’s along with 50 people without the disorder as controls. In the initial testing, they used data from a standard battery of movement tests. This yielded an overall accuracy of more than 92% for distinguishing individuals with Parkinson’s from controls.
The model also showed more than 89% accuracy for distinguishing people with mild Parkinson’s from controls, which the researchers said is probably more relevant for a tool that aims to improve the diagnosis for patients in the earliest stages of disease.
In additional analyses, the researchers found that data from just one movement task, called the cognitive timed-up-and-go, or cogTUG, was enough for a highly accurate Parkinson’s prediction. In the cogTUG, a person stands up, walks a few feet, turns around, and sits down while performing a cognitive task, such as counting backwards from 100 by multiples of three. Data from just this task reduced the accuracy of the models by less than 3%, compared with data from the whole battery of movement tasks.
“These results suggest that sensor data from a single appropriately chosen mobility task may be sufficient to achieve satisfactory performance in differentiating [Parkinson’s] and control participants,” wrote the scientists, who noted the setup achieved “diagnostic accuracy for [Parkinson’s] exceeding that typically achieved by movement disorders experts in a routine clinical setting.”
While the early results are promising, the researchers emphasized that additional work is needed to verify the utility of the setup for diagnosing Parkinson’s in clinical practice.