Predictive Models Could Help Diagnose Early-stage Parkinson’s, Study Finds
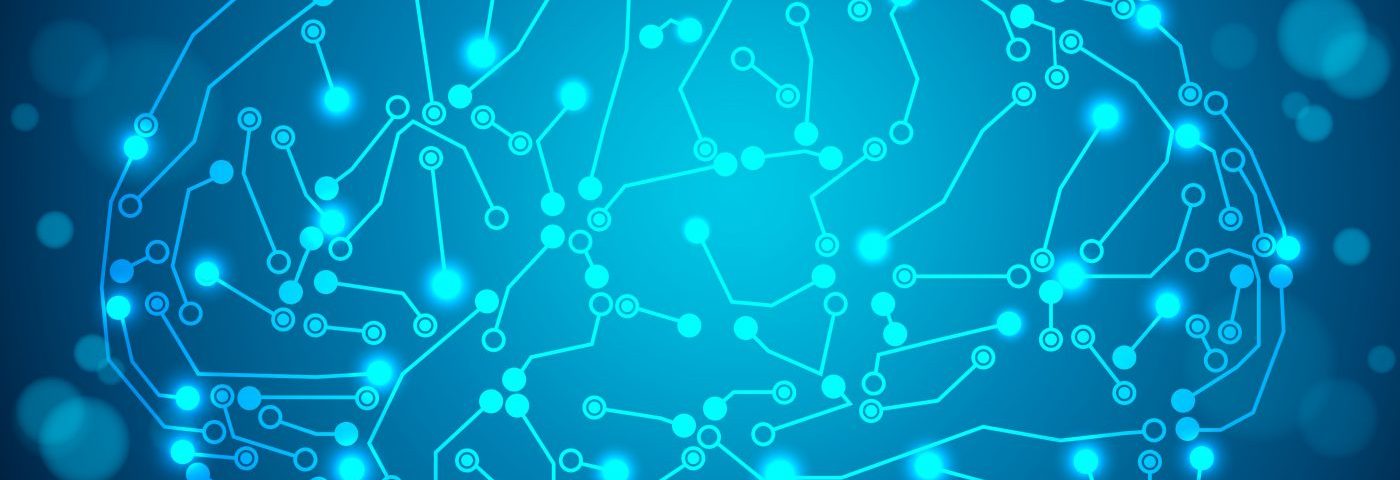
Predictive models can help diagnose Parkinson’s disease in early stages, and could be used to distinguish Parkinson’s from conditions that present similarly, a new study suggests. The findings indicate that losing the sense of smell is particularly significant for predicting Parkinson’s.
The study, “Non-motor Clinical and Biomarker Predictors Enable High Cross-Validated Accuracy Detection of Early PD but Lesser Cross-Validated Accuracy Detection of Scans Without Evidence of Dopaminergic Deficit,” was published in Frontiers in Neurology.
It is widely agreed that non-motor manifestations of Parkinson’s disease typically predate the onset of motor symptoms that are more specifically characteristic of the disease. Identifying Parkinson’s in these very early stages, which is necessary for starting treatment as soon as possible, is a continuous clinical challenge.
A particular problem in this regard is differentiating between Parkinson’s and SWEDD (scans without evidence of dopamine deficit). SWEDD is a disease category in which a person has symptoms that are indicative of Parkinson’s, but they don’t have the changes in dopamine activity in the brain that are characteristic of Parkinson’s. The researchers who authored the new study described SWEDD as “a [Parkinson’s disease] lookalike.”
In the new study, researchers constructed five different predictive models with the dual goals of differentiating between people with early Parkinson’s and people without disease, and between people with early Parkinson’s and people with SWEDD.
Conceptually, these models were constructed by feeding clinical, biological, and demographic data into computers. Then, using specialized algorithms, the computer develops rules for distinguishing between the two relevant groups. The different models used are essentially just different underlying algorithms for this same purpose.
“Every feature used was first proven relevant in the literature. Of those, we allowed each model to pick out which predictors were most important,” study co-author Charles Leger, a PhD candidate at York University in Canada, said in a press release. “No model is guaranteed to provide the best fit. With five models, if you get the same feature that stands out, then you know that particular variable is very important in distinguishing disease. Neurologists could apply one or more of the models to their own data to … distinguish Parkinson’s pathology from pathology masquerading as Parkinson’s.”
The data used for the models was obtained from the Parkinson’s Progression Markers Initiative (PPMI) database, an observational, international clinical study to establish Parkinson’s biomarkers. The specific features analyzed in the models included loss of sense of smell, education, daytime sleepiness, and rapid eye movement (REM) sleep behavior disorder.
In total, data for 295 people with early PD, 43 with SWEDD, and 130 with no evidence of disease were analyzed. In order to be able to test their models, the researchers built them using only a subset of this data. The models were then tested on the remaining data.
The diagnostic accuracy of the models was assessed by calculating the area under the receiver operating characteristic curve (AUC). AUC is a statistical measurement of how well a given model can distinguish between two groups — in this case, early Parkinson’s vs. no disease and early Parkinson’s vs. SWEDD, in the two respective analyses. AUC values can range from 0 to 1; a value closer to 1 indicated better distinguishing accuracy.
All five models performed well at distinguishing early Parkinson’s from no disease; AUC values ranged from 0.86 to 0.928. Distinguishing early Parkinson’s from SWEDD was less definitive, with AUC values between 0.743 and 0.863.
“The discrepancy of model performance between early PD/control and early PD/SWEDD classification is, at least in part, due to the wide range of disorders encompassed by the SWEDD category,” the researchers wrote, noting that some in the field have called for a removal of the term or category SWEDD for this very reason.
Across the models, loss of sense of smell was the most important differentiator of early Parkinson’s in both analyses, with the second-most important being REM sleep behavior disorder. This indicates the importance of these symptoms in identifying early Parkinson’s disease.
Interestingly, daytime sleepiness, age, and education were important for distinguishing early Parkinson’s from SWEDD, but weren’t important for differentiating early Parkinson’s from no disease. Other variables, including levels of Parkinson’s-related biomarkers in the fluid surrounding the brain and spinal cord (cerebrospinal fluid, CSF), namely alpha-synuclein, were more important for distinguishing early Parkinson’s from no disease than from SWEDD. These findings, “warrant further investigation,” the researchers wrote.
Overall, this study supports the use of these models for detecting early Parkinson’s disease.
“These models could be very useful in differentiating patients who may present with Parkinson’s-like symptoms not related to Parkinson’s pathology from patients who actually have the disease,” said study co-author Joseph DeSouza, PhD, a professor at York University.