People with Reduced Smell, Taste Abilities at Risk of Parkinson’s Detected by Machine Learning
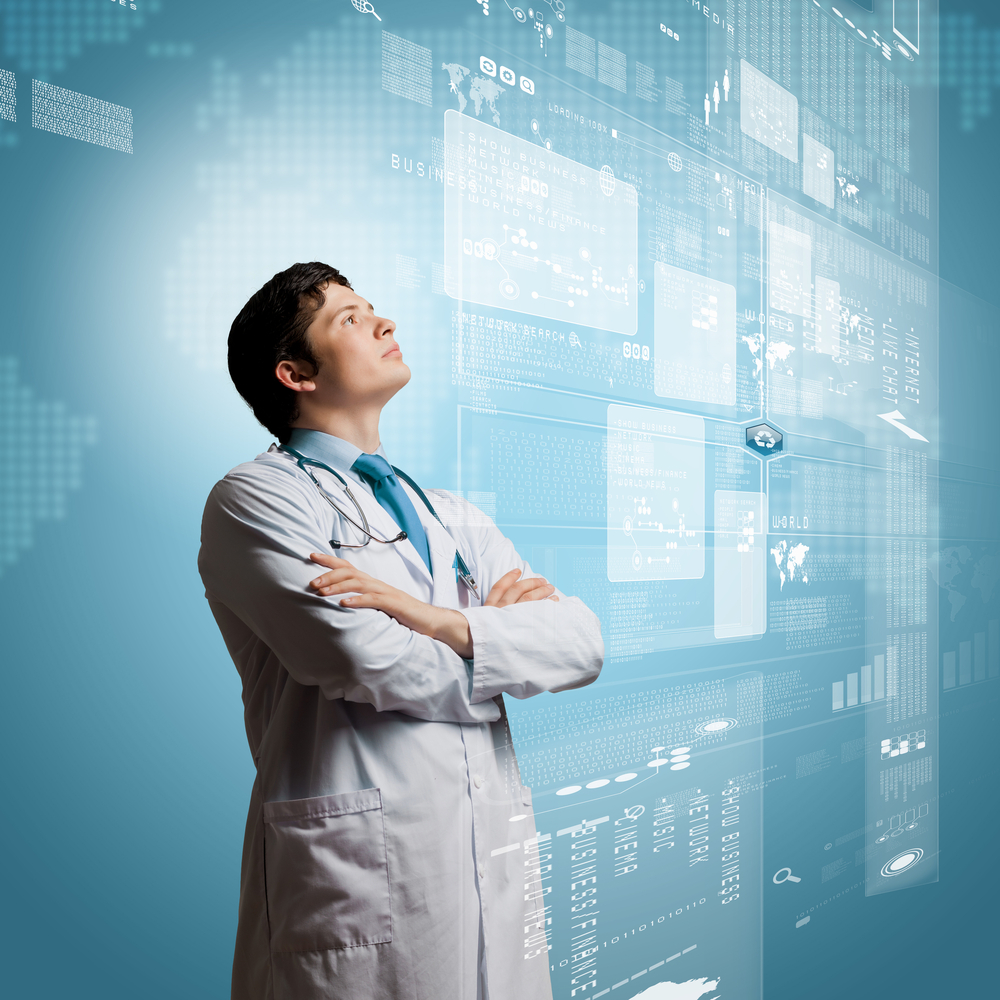
Machine learning identifies individuals with reduced odor and taste sensitivity as people at risk for developing Parkinson’s disease, researchers from Italy report. The approach could be part of a noninvasive method to study early-onset Parkinson’s, the findings suggest.
The study, “Comparative Motor Pre-clinical Assessment in Parkinson’s Disease Using Supervised Machine Learning Approaches,” appeared in the journal Annals of Biomedical Engineering.
Currently, the diagnosis of Parkinson’s is based mainly on its motor symptoms, Specifically, clinicians use scales such as the Movement Disorder Society-sponsored revision of the Unified Parkinson’s Disease Rating Scale (MDS-UPDRS) to determine disease severity. However, the score is subjective and the detection of small changes in motor performance may be challenging.
Nonmotor symptoms such as idiopathic hyposmia (IH) — reduced ability to smell and taste — have been the focus of increased attention due to their potential to anticipate motor manifestations.
However, issues related to their low specificity for Parkinson’s preclude their use to identify individuals with early, or prodromal, disease.
Imaging techniques such as single proton emission computed tomography (SPECT) are currently used to confirm diagnosis, but they require highly skilled staff, and may be invasive, expensive, or not sufficiently specific.
Machine learning, a subset of artificial intelligence, has been used to differentiate Parkinson’s patients from healthy controls regarding the motor ability of their lower limbs. However, no study has applied machine learning algorithms — a list of rules computers follow to solve problems — to people at risk of developing Parkinson’s, specifically those with IH, according to the authors.
So, the research team tested the ability of three machine learning approaches to differentiate lower limb performance in diagnosed Parkinson’s patients, people with IH, and healthy people, or controls (30 subjects each).
Motor performance in four exercises — leg agility, toe tapping, gait and rotation, all part of MDS-UPDRS section III — was measured through wearable sensors called inertial measurement units.
Results showed that all three approaches accurately distinguished patients, people with IH, and controls. Individuals with IH showed an intermediate motor performance between controls and patients.
“Thus, IH subjects can be identifiable as people at risk of developing the pathology in a prodromal phase,” the researchers wrote.
“Further, identification of IH together with changes in motor parameters could be a noninvasive two-step approach to investigate the early onset of [Parkinson’s disease],” they added.
Of note, the findings also indicated that all four exercises were important to classify the participants. According to the scientists, this will encourage researchers to also use tapping exercises in assessments of motor performance, unlike most studies that used gait only.
Among the study’s limitations, the researchers mentioned the lack of correlation with clinical scales, as the analysis did not consider Parkinson’s severity or the level of IH. Future research should have larger sample sizes and analyze upper limb motor performance as well, they advised.