Foundational AI tool identifies Parkinson’s by analyzing eye images
Scientists make RETFound publicly available to help continue research
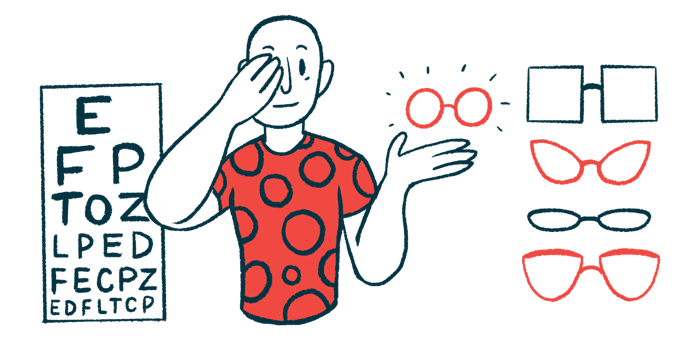
Researchers have developed a new tool, called RETFound, to analyze images of the eyes using artificial intelligence (AI).
The scientists showed this tool can be used to accurately identify eye diseases, and it could even identify other disorders including heart failure and Parkinson’s disease.
“This is another big step towards using AI to reinvent the eye examination for the 21st century … . We show several exemplar conditions where RETFound can be used, but it has the potential to be developed further for hundreds of other sight-threatening eye diseases that we haven’t yet explored,” Pearse Keane, MD, co-author of the study at University College London, said in a press release.
The study, “A foundation model for generalizable disease detection from retinal images,” was published in Nature.
Analyzing images of the retina — the back part of the eye that houses light-sensing cells — is a crucial tool for diagnosing many eye-related disorders. An emerging body of research has suggested that analyses of the retina also could help detect some body-wide disorders including Parkinson’s.
AI to analyze images of eye’s retina
In the study, researchers set out to construct a new AI model that could be used to analyze images of the retina.
Traditionally, AI works by feeding a computer a significant amount of data, alongside a set of mathematical algorithms that the computer uses to learn and identify patterns. For AI-based analyses of eye structures, prior research has largely relied on images that have been labeled by human experts.
Here the researchers instead wanted to create a foundational model to analyze the retina. Simplistically, this involves feeding the computer a huge quantity of unlabeled data, and letting the computer find patterns amongst the data without further guidance. Then, the model can be applied to a specific task, such as identifying people with a particular disease.
A well-known example of a foundational AI model is ChatGPT, which is trained on large quantities of unlabeled human-written text.
“A foundation model is defined as a large AI model trained on a vast quantity of unlabelled data at scale resulting in a model that can be adapted to a wide range of downstream tasks,” the researchers wrote.
“Over the course of millions of images, the model somehow learns what a retina looks like and what all the features of a retina are,” Keane said in a separate Nature news story.
The fact that this type of model can use unlabeled data is important, as obtaining high-quality labels for medical data is an expensive and time-consuming task.
The scientists created their model, called RETFound, by training a computer on hundreds of thousands of images of the retina, taken with one of two technologies: color fundus photography or optical coherence tomography. They then tested how well the model could identify various disorders.
In order to calculate the models accuracy, the researchers used a statistical measure called the area under the receiver operating characteristic curve or AUROC. This is a statistical measure of how well a test can distinguish between two groups, e.g., people with and without a disease. AUROC values can range from 0 to 1, with higher numbers reflecting a better ability to differentiate.
Results showed that RETFound provided high accuracy for identifying eye disorders. For example, in identifying diabetic retinopathy (a form of eye disease associated with diabetes), the AUROC was up to 0.943.
RETFound also identified other disorders including Parkinson’s, heart failure
The model also showed good accuracy for identifying several non-eye disorders. For heart failure, the model had an AUROC of 0.794, while for Parkinson’s it was 0.669.
The researchers emphasized that, while the model showed good accuracy, there is still room for improvement — for example, they noted the model doesn’t currently include data on demographics or eyesight, which could help improve accuracy. The model also was trained based on data from people in the U.K., so further work may be needed to ensure it can accurately reflect a global population.
To help spur research with RETFound, the researchers have made the tool publicly available for other scientists.
“By making RETFound publicly available, we hope to accelerate the progress of AI in medicine by enabling researchers to use our large dataset to design models for use in their own institutions or to explore alternative downstream applications,” they wrote.
Keane noted in the Nature article that other researchers “could potentially take this algorithm and fine-tune it, using data from their own country to have something that’s more optimized for their use.”