New AI tool may predict different structures of amyloid fibrils: Study
Misfolded proteins are 'polymorphic,' making predicting their structure difficult
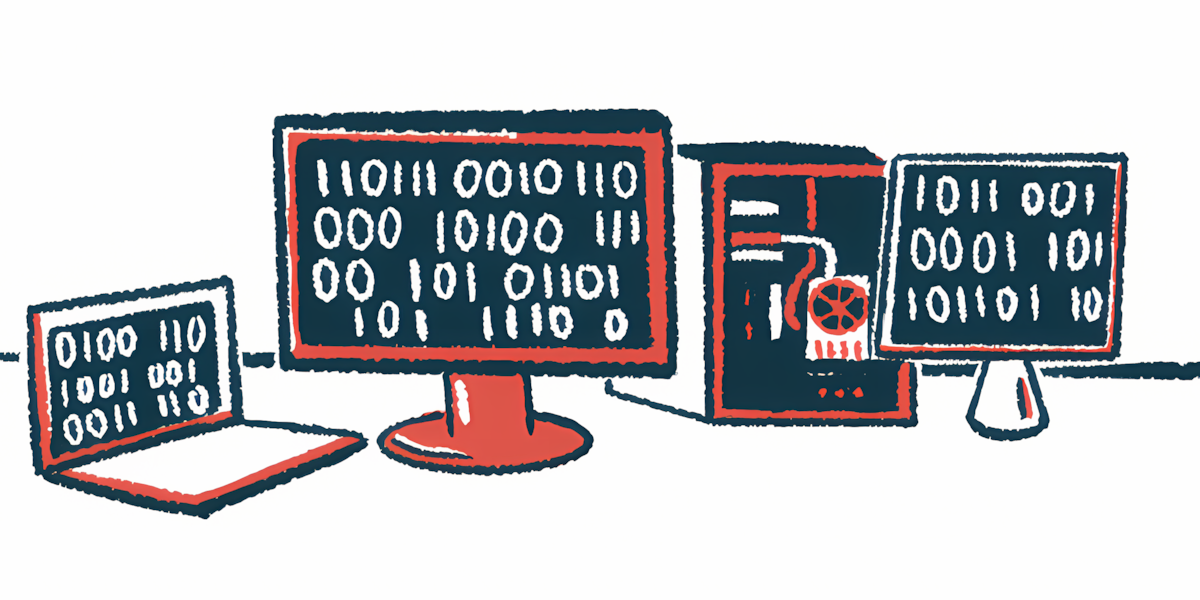
A new artificial intelligence (AI) tool may help predict the structure of amyloid fibrils, that is, misfolded protein fibers that accumulate in the brain of people with neurodegenerative diseases such as Parkinson’s.
Called Ribbonfold, the tool is designed to address the complex and variable structures of misfolded proteins by including “constraints” appropriate for detecting their ribbonlike features.
“The ability to predict amyloid polymorphs [variants] efficiently may guide future breakthroughs in preventing harmful protein aggregation, a crucial step toward tackling some of the world’s most pressing neurodegenerative challenges,” Peter G. Wolynes, PhD, professor at Rice University, co-director of the Center for Theoretical Biological Physics, and co-author of the study, said in a university news story. The study, “Generating the polymorph landscapes of amyloid fibrils using AI: RibbonFold,” was published in the Proceedings of the National Academy of Science.
A common feature of neurodegenerative diseases such as Parkinson’s and Alzheimer’s is the accumulation of misfolded proteins into specific types of insoluble, fibrous clumps called amyloid fibrils that are thought to contribute to nerve cell death in these diseases. In Parkinson’s, toxic aggregates called Lewy bodies form that are made mostly of alpha-synuclein, while clumps of amyloid-beta and tau are linked to Alzheimer’s.
The formation of amyloid fibrils involves complex mechanisms and structural polymorphism, meaning the same protein sequence can form fibrils with distinct shapes. While structure prediction tools like Alphafold can predict the structures of correctly folded, functional proteins based on their sequences, the variable and aggregated nature of amyloid fibrils makes predicting their structure challenging. Alphafold is an AI tool developed by Google DeepMind that can predict the 3D shape of a protein just by knowing its amino acid sequence, that is, the biological instructions for making a protein.
How is Ribbonfold able to predict the structure of amyloid fibrils?
Ribbonfold was adapted from Alphafold2, the second and improved version of the AlphaFold system, and includes a feature called “parallel in-register constraints,” which means that multiple protein chains line up side by side in the same direction, with matching amino acids in the same positions. This alignment helps make the protein clumps more stable and less soluble, while still allowing different kinds of interactions between the chains.
The model was trained using available structural data on amyloid fibrils and was validated for other known fibril structures that weren’t included in the training. Additionally, Ribbonfold was tested using four recently released sequences. It was found to outperform existing AI tools at predicting amyloid fibril structures and suggested that fibrils may begin in one structural form and shift into more insoluble structures over time, contributing to disease progression.
“Our method shows that stable polymorphs will likely win out over time by being more insoluble than other forms, explaining the late onset of symptoms. This idea could reshape how researchers approach neurodegenerative disease treatment,” Wolynes said.
By offering an accurate method for analyzing the structure of amyloid fibrils, the new AI tool may contribute to developing new drugs that target the most disease-relevant amyloid fibril structures.
“The ability to predict amyloid polymorphs efficiently may guide future breakthroughs in preventing harmful protein aggregation, a crucial step toward tackling some of the world’s most pressing neurodegenerative challenges,” Wolynes said.