AI algorithm uses smartwatch data to predict Parkinson’s emotions
Experimental system measures physiological changes
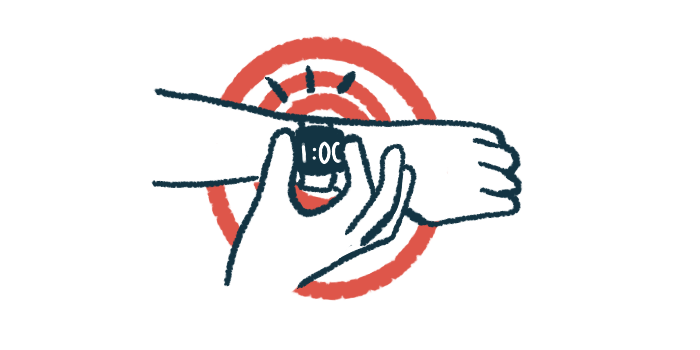
Researchers have developed an algorithm that uses artificial intelligence (AI) to recognize the emotional states of people with Parkinson’s disease based on physiological data collected from a smartwatch.
The approach could ultimately be used to help clinicians accurately track emotions in their patients and guide treatment decisions, the researchers said, noting that the preliminary findings “warrant confirmation from trials on larger samples” as “there are open issues to be deepened in future work.”
Their study, “Supervised learning for automatic emotion recognition in Parkinson’s disease through smartwatch signals,” was published in Expert Systems With Applications.
While Parkinson’s disease is best recognized by its hallmark motor symptoms, the neurodegenerative condition comes with a wide range of nonmotor symptoms. Neuropsychiatric and mood changes, including anxiety, depression, and apathy, are common among Parkinson’s patients.
However, many of these psychological symptoms are highly subjective, and it is not easy to measure them and monitor them in the clinic.
AI algorithm studies haven’t focused on emotions in Parkinson’s
One possible way to monitor a person’s emotional state is to measure physiological changes that correspond with emotion, such as a quickening heart rate or rising body temperature. These changes are related to the function of the autonomic nervous system, which is involved in mediating a range of involuntary bodily functions and is commonly dysregulated in Parkinson’s.
Automatic emotion recognition based on these autonomic changes is an approach that’s been well studied in healthy people, but has not been thoroughly evaluated in Parkinson’s. The scientists set out to understand how it might apply to people with the neurodegenerative disease.
Eleven people with mild to moderate Parkinson’s disease and eight people without neurological disease were asked to watch a series of video clips designed to arouse a variety of different emotions, both positive and negative.
Each subject wore a smartwatch that collected physiological data like heart rate, temperature, and the skin’s electrical conductance. Participants were also asked to report on their emotional state when watching each video.
The scientists then looked for an AI-based approach to predicting a person’s emotional state based on data collected from the smartwatch. They compared a few different types of algorithms.
.Results showed AI was able to identify whether individuals were feeling positive or negative emotions (valence), and how strongly they felt them (level of arousal), with more than 90% accuracy for both Parkinson’s patients and people without Parkinson’s.
Predicting type, intensity of emotion
One algorithm performed significantly better than the others. The most important features used in its prediction were skin temperature and galvanic skin response (electrical activity in the skin), which is known to be important for emotional recognition in healthy people.
While overall accuracy was high, it was more difficult for the algorithm to predict emotional valence than arousal state, the researchers said.
Autonomic dysfunction and motor symptoms in Parkinson’s could lower or cause variability in the autonomic response to a felt emotion, making it more difficult to see patterns in physiological responses, they noted.
That’s one reason it is important for the algorithm to learn how to make its predictions using data from Parkinson’s patients rather than from only people in the general population, the researchers said. “It is possible to recognize emotions in these people by low-cost, widely acceptable and usable methods with high accuracy,” they wrote.
“This finding is of great significance because it provides a prerequisite for the use of these systems in current clinical care for the management of [people with Parkinson’s disease], allowing to discriminate the quality of their emotions (regardless of their intensity) and thus also guide therapeutic decisions to improve the quality of life of both patients and caregivers,” the researchers wrote.
More studies are needed to test and validate the approach in larger groups of patients, and there’s a need to develop algorithms capable of recognizing more complex emotional states such as anger or depression, according to the scientists.
The researchers said a follow-up publication will analyze in more detail the possible clinical significance of the results observed in the paper.