Voice Analysis Has Prognostic Potential In Parkinson’s, Study Contends
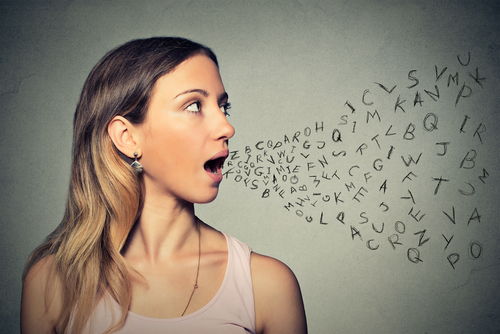
pathdoc/Shutterstock
Computer-based analyses of the voice could be used for at-home monitoring of symptom severity in people with Parkinson’s disease, a small proof-of-concept study suggests.
The study, “Prediction and Estimation of Parkinson’s Disease Severity Based on Voice Signal,” was published in the Journal of Voice.
Dopaminergic therapies — treatments that increase dopamine signaling in the brain, including levodopa and its derivatives — are a mainstay of treatment for Parkinson’s disease. While such treatment can effectively mitigate symptoms, its efficacy typically decreases as the disease progresses. Also, medications are typically short-acting, resulting in “on” periods, during which the medication effectively controls symptoms, and “off” periods, when symptoms return.
Because of this variation, close monitoring is important for providing optimal treatment for Parkinson’s. However, this kind of detailed monitoring isn’t really feasible in a traditional hospital model.
“In normal conditions, the patients meet the doctor every 3−6 months,” wrote the authors of the new study. “Considering the several months which pass between visits and the few minutes available to summarize how the patient feels, and also bearing in mind that the patient suffers from neurological disease and in most cases is an elderly person, it is impossible to share all the relevant information about all of the symptoms and other complications of the disease.”
Non-invasive methods for objectively monitoring Parkinson’s that can be applied easily at home could be of great utility in Parkinson’s care.
Speech abnormalities — such as hoarseness, slurring, and trouble modulating volume or tone — are common in Parkinson’s disease, resulting from problems like impaired facial muscle function. Because of this, vocal analysis may be a useful tool for diagnosing and/or monitoring Parkinson’s.
In the new study, researchers analyzed voice recordings from 27 people with Parkinson’s (age range 50–78 years). For each individual, five recordings were taken at five intervals: during an “off” period (at least three hours after the last dose of levodopa), and 30, 60, 120, and 180 minutes after taking levodopa.
The recordings themselves were of participants making vowel sounds (“a,” “e,” etc.).
At the same time intervals, patients’ motor symptoms were assessed using the motor-specific part of the Unified Parkinson’s Disease Rating Scale (UPDRS).
Researchers then used machine learning to find associations between features of the vocal recordings and motor UPDRS scores. Conceptually, this involved feeding the data into a computer, then allowing the computer to design algorithms to predict UPDRS scores based on the vocal parameters. Different algorithms were developed by giving the computer different rules and strategies with which to work.
The researchers used 80% of the data collected to design these algorithms; the remaining data was used to validate and test the algorithms.
To assess the effectiveness of the developed algorithms, the researchers calculated multiple statistical parameters, most notably the mean absolute error (MAE). As its name suggests, MAE is a measurement of how much error is in a sample, on average — in this case, the average difference between predicted UPDRS scores (based on the vocal recordings) and the actual scores (based on expert assessment). Broadly, the lower the MAE, the better the algorithm.
By this metric, the best-performing algorithm’s MAE was around 4–5, with some values as low as 1.8. For context, the total possible score ranges from 0–108, and previous research has suggested that this amount of variation is about the same as what is seen with different physicians doing the rating. As such, the scores based on vocal prediction are likely to be reflective of actual scores.
“Based on the research methodology presented, it is possible to automate the assessment of the patient’s condition on the UPDRS-III scale,” the researchers concluded.
This provides a proof-of-concept for the development of this type of tool, which could be expanded to develop monitoring systems for Parkinson’s that could be used easily for routine monitoring at home.