Smartphone App Can Identify Early Symptoms of Motor Disorders, Study Reports
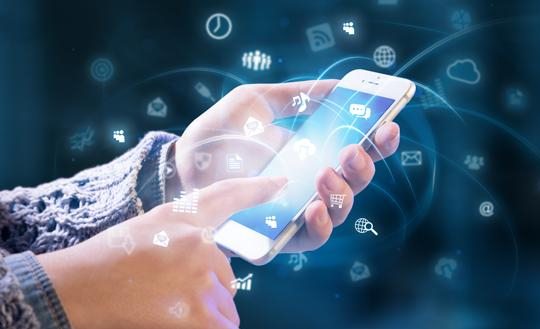
A smartphone application using a touch-based model is able to accurately identify early signs of motor disorders, according to a new study.
The app, developed for patients with Huntington’s disease, is also intended for people with Parkinson’s and other neurological disorders.
The study, “A smartphone application for automated decision support in cognitive task based evaluation of central nervous system motor disorders,” was published in the IEEE Journal of Biomedical and Health Informatics.
Increasingly used for diverse medical practices, these applications employ sensors and multimedia capabilities to provide information about different parts of the body.
The challenging diagnosis of central nervous system motor disorders, such as Parkinson’s and Huntington’s, have led to the development of computational intelligence systems using mobile apps. In Parkinson’s research, wearable sensor technology was used to detect postural and hand tremors, as well as dyskinesia — involuntary, jerky movements — and impaired speech articulation and production.
Most apps that were supported by clinical trials “used a combination of smartphone and wearable sensors, and included the assessment of subjects’ gait or posture abnormalities,” the researchers wrote.
Subsequently, a team from Kaunas University of Technology, in Lithuania, and Silesian University of Technology, in Poland, developed a new machine learning method for automated evaluation of patients with neurological disorders using finger-tapping tests and cognitive tasks.
The proposed method was designed to provide diagnosis in an easy and efficient way. Using a mobile app, patients can perform tests remotely, or with help from healthcare professionals or family members. Readings from sensors in the mobile devices were then evaluated by computational intelligence models.
The mobile app gathers information from three simple tasks, in which the patients have to touch the screen with one or various fingers as close as possible to the indicated targets. Patient response times and images representing the trajectories of their fingers over the screen are obtained.
Compared with healthy individuals, patients with motor disorders do the tasks slower and less precisely, the scientists said. Larger distances between touched points and targets correlate with the severity of hand tremor, while response time is associated with the ability to conduct voluntary movements.
The investigators recommend using mobile devices with high resolution displays, preferably those with big screens, which may be necessary for patients with advanced motor disorders. Besides Huntington’s and Parkinson’s, the model is easily adaptable to neurodegenerative diseases such as Alzheimer’s, or conditions such as mild cognitive impairment, the team said.
The model was implemented as part of the Neural Impairment Test Suite mobile app for Android OS, which is available on Google Play.
In the first of the 3 tasks, circles of a particular color are randomly generated on the screen; the target circle is marked by a black contour, and the goal is to touch each object as close to the center and as quickly as possible.
In the second task, 7 circles of different colors are presented and a text label indicates which circle is to be touched; if no touches are registered, the screen is automatically redrawn. The patient is instructed to touch each object 5 times.
As for the third task, 2, 3 or 6 circles are presented in parallel. When presented with 6 circles, the patient needs to use both hands, each for a separate color.
Huntington’s disease patients and healthy controls — 11 each — collaborated in the research. A total of 1,928 records were obtained, as the participants were asked to repeat the tasks once or twice a week to evaluate disease progression. The data can be used to predict whether the patient is at high risk for fast development of Huntington’s symptoms, as well as to distinguish patients from healthy individuals. The participants’ average reaction time is calculated in different age groups: up to 20 years, from 20 to 40 years, from 40 to 60 years, and over 60 years.
The tests revealed that patients and controls significantly differed in the distance between target and observed positions, movement speed, and response time. The system showed an 86.4% accuracy in recognizing early symptoms of motor disorders.
“This result clearly shows that the proposed app can be a useful support in automated medical examinations of patients with central nervous system motor disorders,” the researchers wrote. “We hope that our research can lead us to make the use of mobile devices in medical examinations simple and useful in diagnosis of various disorders of similar type.”
Among their future plans, the team is considering including additional features to evaluate cognitive level, as well as motor and non-motor symptoms in Parkinson’s disease. The scientists also recommend developing more tasks for higher accuracy.