Predicting Whose Parkinson’s Will Progress to Speed Therapy Trials
Machine-learning tool supporting one-year studies into disease-modifying agents
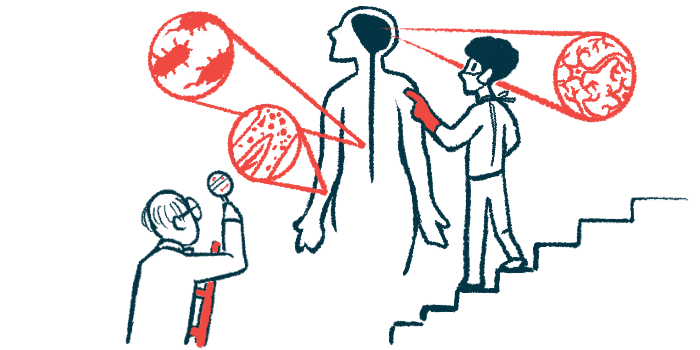
A machine-learning tool that combines genetic and clinical data can distinguish, with a high level of accuracy, between fast- and slow-progressing Parkinson’s patients, a study reported.
The tool could be used to predict those patients who are more likely to progress over the short term — and who could be the target population for clinical trials of therapies aiming to slow disease progression.
“If clinicians are able to enroll in trials only those patients predicted to progress, they can get much faster results and move this field along more quickly,” Ali Torkamani, PhD, the study’s senior author and an assistant professor of molecular and experimental medicine at the Scripps Research Translational Institute, said in an institute press release.
“Right now, [Parkinson’s] clinical trials are large and tend to take two to three years. We’re hoping to empower smaller trials that are on the order of a one-year time frame,” Torkamani added.
Parkinson’s progression is highly variable among patients, hampering trials
The study, “Genetically-informed prediction of short-term Parkinson’s disease progression,” was published in npj Parkinson’s Disease. The work was supported by Takeda Development Center Americas, which is involved in the development of potential Parkinson’s treatments, and by the National Institutes of Health.
Parkinson’s is a progressive disorder, meaning it tends to worsen as time goes on. Available disease treatments can ease the symptoms of Parkinson’s, but none have been proven to alter its progression.
The rate at which Parkinson’s progresses varies considerably from person to person, and many patients do not experience symptom worsening over a single year, the study noted.
This can pose a problem for clinical trials testing experimental therapies that aim to slow progression: it can be hard to detect a therapy’s potential effectiveness amid the noise of patient-to-patient variability.
“These issues result in expensive and time-consuming clinical trials prone to failure,” the researchers wrote.
Torkamani and colleagues at Scripps Research and the Takeda center set out to create a tool that would identify those patients more likely to experience disease progression over a 12-month time frame.
Their ultimate goal is “shortening the duration of clinical trials by selectively recruiting subjects who are destined to be short-term progressors,” the researchers wrote, so that meaningful treatment effects might be easier to record.
To create this tool, the researchers used a machine learning approach. Specifically, they used a form of machine learning called meta-prediction, which the team noted is well-suited for accounting for the way that motor and non-motor symptoms can progress in distinct ways.
Essentially, the scientists fed data into a computer program, alongside information about a patient’s progression or lack of progression, defined by increasing (worsening) scores on the MDS-UPDRS, a measure of Parkinson’s severity based on a rating of symptoms covered in its four parts.
Progressors and non-progressors were defined based on the slope of their medication-adjusted MDS-UPDRS values for each time period: from study’s entry to one, two, and three years later.
The computer then used a prespecified set of mathematical algorithms to “learn” what features in these data were predictive of disease progression at each time period.
Tool reported to ‘well predict’ patients with progression over one year
Information given the model included demographic features like age, sex, and educational status, standardized measures of symptom severity like initial MDS-UPDRS scores, imaging data from brain scans, treatment use, and genetic data.
“This model worked by combining different aspects of comprehensive disease profiling,” Torkamani said.
Computers were “trained” using data from 529 patients participating in the Parkinson’s Progression Markers Initiative (PPMI). Finally, the computer-created models were tested mainly using data from a separate group of 350 patients taking part in the Parkinson’s Disease Biomarkers Program (PDBP).
Among the PPMI group, 64% of patients were identified as “progressors,” as were 48% of those in the PDBP group.
A statistical test called area under the curve, or AUC, was used to determine the program’s accuracy in identifying progressors. This test measures how well a model can tell the difference between two groups — in this case, patients who did or did not progress over the follow-up periods. AUC values can range from 0 to 1, with 0 indicating that 100% of its predictions are wrong and 1 that 100% of its predictions are correct.
This machine learning model was able to identify Parkinson’s progressors with an AUC of up to 0.77 at one year of follow-up, the study reported.
“We demonstrate that short-term [Parkinson’s disease] progression can be well predicted with a combination of survey-based, neuroimaging, physician examination, and genetic predictors in a meta-prediction framework,” the researchers wrote. “These predictions may be useful in accelerating the identification of [Parkinson’s] disease modifying agents.”
In further analyses, the researchers tested how the model’s predictive power changed when certain data were removed from its training. The most substantial loss in accuracy occurred when genetic data was removed, they found.
This suggests that “genetic risk factors were the most powerful predictor” of progression, Torkamani said, “but other factors were important to include as well.”
The Scripps Research scientists now plan to evaluate whether their model can be used to predict changes in specific Parkinson’s symptoms, including whether genetic markers might indicate a likelihood of developing disease-related psychosis or depression.