Algorithm Accurately Predicts Risk of Cognitive Decline in Parkinson’s
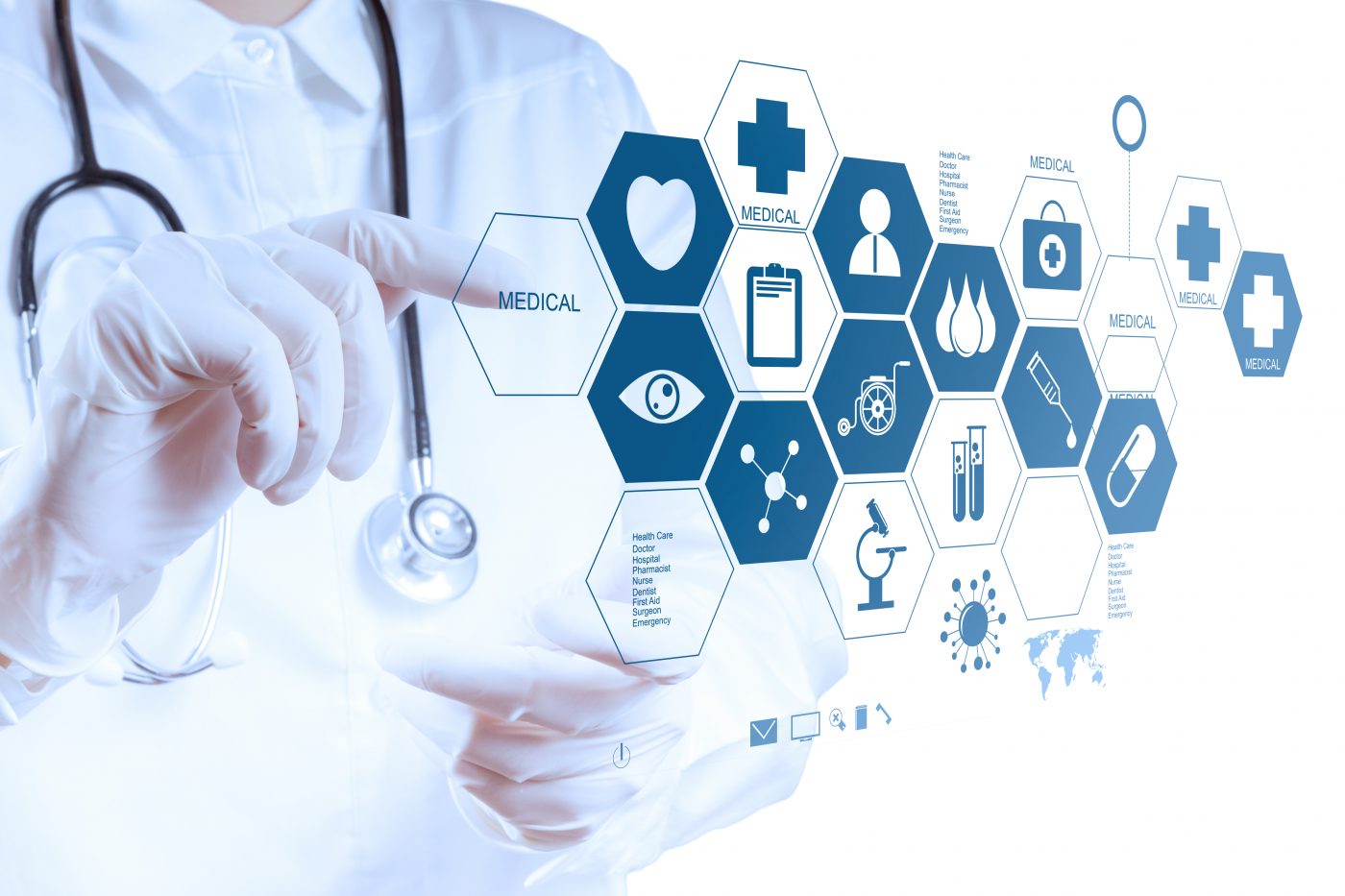
A new algorithm can accurately predict cognitive decline in Parkinson’s disease patients within 10 years of disease onset, a study shows.
The study, “Prediction of cognition in Parkinson’s disease with a clinical–genetic score: a longitudinal analysis of nine cohorts,” was published in the journal The Lancet Neurology.
Cognitive decline is a major manifestation of disease progression in patients with Parkinson’s disease, and the ability to predict which patients will develop cognitive decline earlier can help recruitment and classification of patients into the right clinical trials. Currently, while there is a plethora of drugs that target motor defects in Parkinson’s disease patients, they do not have an effect on cognitive decline.
There are known risk factors that predispose Parkinson’s disease patients to developing cognitive decline. Age of onset, depression, education, disease severity, motor score, cognitive score at baseline, and mutations in the β-glucocerebrosidase gene are just some of the factors that have been associated with risk of cognitive decline.
So, a group of multinational collaborative investigators, part of the International Genetics of Parkinson’s Disease Progression Consortium, developed a clinical-genetic score that can predict cognitive impairment in Parkinson’s disease patients, There currently is no widely applicable assessment tools available to predict cognitive decline.
To construct the predictive tool, researchers analyzed cognitive and other data from 1,350 patients with Parkinson’s disease from six different studies. Patients had undergone 5,165 physician visits over the course of 12.8 years.
The team looked at multiple known risk factors for each patient, including age at onset, baseline MMSE (Mini-Mental State Exam), years of education, motor exam score, sex, depression, and β-glucocerebrosidase (GBA) mutation status. These factors then were included in the algorithm to create a prediction model.
Risk was divided into four quartiles. Patients who scored the lowest (quartile 4) were at the highest risk of cognitive decline within 10 years of disease onset. In fact, these patients had a 18.4-fold higher risk compared to patients in quartile 1, the lowest quartile.
Furthermore, patients in the highest quartile were at a 21.9-fold greater risk for developing dementia compared to patients in quartile 1. Interestingly, 98.9 percent of patients in quartile 1 did not develop dementia in the 10 years following diagnosis versus 48.3 percent of patients in the highest quartile.
Sensitivity of a test is the ability to correctly identify patients with the disease while the specificity is the ability to correctly identify those without the disease. The prediction generated by this algorithm was highly accurate with 87 percent sensitivity and 72 percent specificity of the model for the prediction of cognitive decline. Similar results were obtained for specificity and sensitivity in prediction of dementia.
This prediction tool was tested in 10,000 training and test sets of randomly generated patient populations, results of which showed excellent stability of the model.
The authors concluded: “Our predictive algorithm provides a potential test for future cognitive health or impairment in patients with Parkinson’s disease. This model could improve trials of cognitive interventions and inform on prognosis.”