EEG Plus Machine-learning Identifies Mild Cognitive Impairment, Study Finds
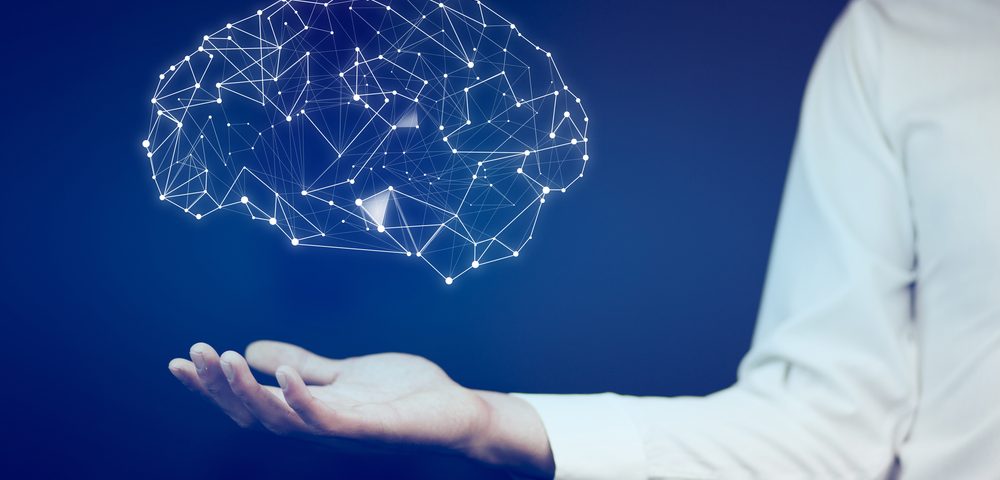
Using non-invasive, dry-electroencephalography (EEG) and machine-learning computer algorithms, researchers were able to distinguish between Parkinson’s disease patients with and without mild cognitive impairments with 80% accuracy, early results of a study found.
Follow-up assessments will be conducted after 12 months to validate the method’s ability to predict cognitive impairment in this patient population.
The results were presented in a poster, “The Identification of Mild Cognitive Impairment in Parkinson’s disease using EEG and Machine Learning, “at the Alzheimer’s Association International Conference 2020. The poster abstract was published in the journal Alzheimer’s & Dementia.
Cognitive impairment is a common symptom of Parkinson’s disease characterized by difficulties in executive function, attention, vision, word-finding, and problems with learning and remembering information.
Cognitive changes can range from Parkinson’s disease mild cognitive impairment (PD-MCI) to Parkinson’s disease dementia (PDD). Moreover, the presence of PD-MCI is associated with an increased risk of dementia. As such, it is essential to identify patients with MCI as early as possible.
EEG is a fast, inexpensive, non-invasive method that measures the brain’s electrical activity and has been used to predict MCI outcomes in people with Alzheimer’s disease.
However, how EEG patterns correlate with MCI in Parkinson’s patients is unclear.
Now, researchers based at the Queen’s University Belfast in Northern Ireland used EEG to evaluate cognitive impairment in people with Parkinson’s over 12 months.
The team used a so-called “dry-EEG” mobile headset, which does not require the use of semi-dry saline or gels to create contact between sensors and the scalp, making it faster to use and more comfortable to wear.
The study included 50 people with Parkinson’s and 50 age- and sex-matched healthy controls. Before the study (baseline), all participants completed a series of neuropsychological and EEG tasks, which included assessments in attention, language, memory, vision, and executive function. EEG while resting also was recorded.
Various statistical methods, as well as machine-learning algorithms, were applied to analyze the data.
The team has now presented the findings relative to the baseline results. Identical follow-up assessments at 12 months will be conducted.
Of those in the Parkinson’s disease group, 52% fulfilled the criteria for MCI. No significant differences were found in age, education, or IQ measures before MCI onset.
Based on the EEG and neuropsychological tests, the machine-learning algorithms were able to distinguish between Parkinson’s patients without cognitive impairment, those with PD-MCI, and healthy controls with an 80% accuracy.
This method’s stability and ability to predict cognitive status at 12 months will be investigated at a follow-up assessment.
“Cognitive impairment is prevalent in [Parkinson’s disease]” the researchers concluded. “Our findings show that it is feasible to use dry-EEG to profile cognition in [Parkinson’s disease] and can differentially discriminate between cognitively normal and cognitively impaired [Parkinson’s disease] patients at an early stage.”