Way of Diagnosing Parkinson’s by ‘Skin Smell’ Test Detailed in Study
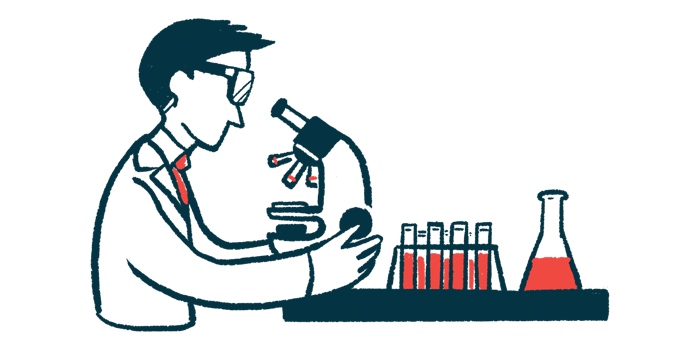
Diagnosing Parkinson’s disease might be a matter of using artificial intelligence to “smell” patients’ skin, scientists in China reported.
The artificial intelligence smell system they developed is detailed in study, “Artificial Intelligent Olfactory System for the Diagnosis of Parkinson’s Disease,” published in ACS Omega.
No single test can now diagnose Parkinson’s; the disease’s is typically determined based on a telltale combination of clinical symptoms and signs. Diagnostic journeys are often complicated, and many patients don’t get a definitive diagnosis until their disease has markedly progressed.
As such, there is a continual need to find ways to make Parkinson’s diagnoses earlier and more accurately.
Recent research suggests that Parkinson’s patients exhibit differences in certain volatile organic compounds (VOCs) in the sebum, the oil that covers the surface of the skin. VOCs are molecules able to easily evaporate out into the air, meaning they are easily detected by the nose.
Scientists at Zhejiang University devised a system to diagnose Parkinson’s by “smelling” sebum to determine the concentration of VOCs.
Put simply, their method involves a sophisticated chemical analysis — fast gas chromatography combined with a surface acoustic wave sensor — to identify the VOCs in a sample. Data gathered through this analysis is then fed into a machine learning algorithm.
For machine learning, the researchers used sebum samples from 31 people with Parkinson’s, as well as 32 individuals without the disease (controls). Based on specific mathematical constraints, a computer devises algorithms to distinguish Parkinson’s patients from controls.
The researchers then tested the algorithms on an additional 12 Parkinson’s patients and an equal number of controls.
Results showed that three VOCs, called octanal, hexyl acetate, and perillic aldehyde, were markedly different between patients and controls. Algorithms based on these three VOCs could diagnose Parkinson’s with an overall accuracy of 70.8%, the researchers calculated.
More specifically, the model’s sensitivity (true-positive rate) was 91.7%, although its specificity (true-negative rate) was 50%. The team also showed that using different mathematical strategies to generate algorithms during machine learning could improve the specificity.
The researchers concluded that their smell test system “is a fast, easy-to-use, and noninvasive method that can be widely used in hospitals, clinics, and homes to screen, diagnose, and monitor [Parkinson’s] treatment.”