B-SOiD Algorithm May Make it Easier to Study Behavior
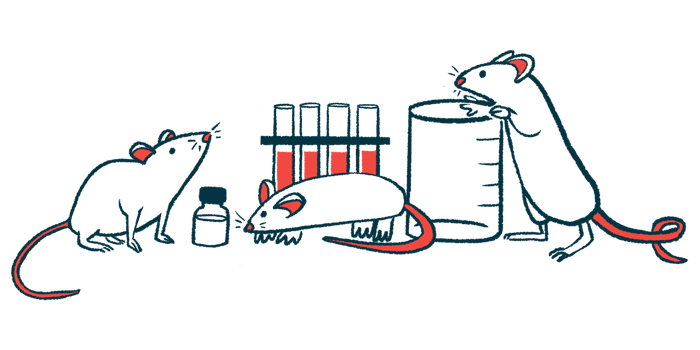
A novel machine learning algorithm called B-SOiD quickly and efficiently analyzes animal behaviors, and may be useful in assessing movement disorders in people with Parkinson’s disease.
Researchers described their findings in the study, “B-SOiD, an open-source unsupervised algorithm for identification and fast prediction of behaviors,” published in Nature Communications.
Doing research on behavior is very difficult because of the complexity of behaviors. Even mice and rats, often used in labs for their relative simplicity, engage in a wide variety of behaviors throughout the day as they scurry about, groom themselves, and eat.
Traditional research on behavior typically has relied on one of two strategies. One is training animals to do a specific task (e.g., pushing a lever), then assessing that behavior. But this has the obvious limitation of the behavior being a construct of the experiment.
Using another approach, some researchers have studied behavior by assessing videos of animals over time and making detailed notes. While this method is better at capturing naturalistic behaviors, it has several drawbacks: it is time- and work-intensive, and is prone to human errors and inconsistencies.
Now, a pair of scientists at Carnegie Mellon University (CMU) in Pittsburgh, Pennsylvania, have built on prior research to create a machine learning algorithm, B-SOiD, that aims to overcome these problems by removing the human component.
Conceptually, the idea is that the algorithm is fed video of an animal; then, the computer identifies the poses of the animal’s body as it goes about its day. These poses over time are then translated into discreet behaviors. For example, when a mouse raises its paws to wipe at its snout (a series of poses), B-SOiD can identify the behavior as grooming.
Simply put, the algorithm aims to collect all the data that might be recorded by a scientist watching the video and taking notes, but without needing the scientist there. As such, it saves researchers’ time and, because the analysis is done by a computer, it can be done very fast. The algorithm can process 100,000 frames per minute on a typical laptop, according to the researchers.
“It removes user bias and, more importantly, removes the time cost and arduous work. We can accurately process hours of data in a matter of minutes,” Eric Yttri, PhD, a professor at CMU and study co-author, said in a press release.
Yttri and Alex Hsu, a biological sciences PhD candidate in his lab, demonstrated the utility of B-SOiD using recordings of mice. Using different animals, the team showed the algorithm could identify behaviors regardless of the color or size of the mouse.
They also showed that B-SOiD could identify similar, but discreet behaviors. For example, the algorithm identified three distinct and well-documented mouse grooming behaviors (paw/face groom, head groom, and body lick). These behaviors were all identified as distinct from itching behavior.
The researchers noted that the algorithm’s ability to “both generalize and differentiate” is crucial in the study of behaviors. Further experiments indicated that B-SOiD could identify changes in behavior in rats with damage to part of the brain called the basal ganglia, the dysfunction of which is implicated in Huntington’s disease and obsessive-compulsive disorder (OCD).
The scientists also showed that the algorithm worked similarly using video of cameras placed at different angles, which is important for generalizability. They have made the algorithm openly available to other researchers.
“The open source package provides a platform that is accessible to biologists without extensive coding knowledge nor computational resources,” they wrote.
The team is hopeful the algorithm may allow for the development of health assessments for people. They already have begun to use B-SOiD to study movement in people with Parkinson’s.
“We are beginning to see if this can be used as part of an objective test by a doctor to show how far a patient’s disease has progressed. The hope is that a patient anywhere in the world would be diagnosed with one standardized metric,” Yttri said.