AI tools may help detect, diagnose Parkinson’s at early stages: Study
Advanced imaging may ID disease before motor symptom onset
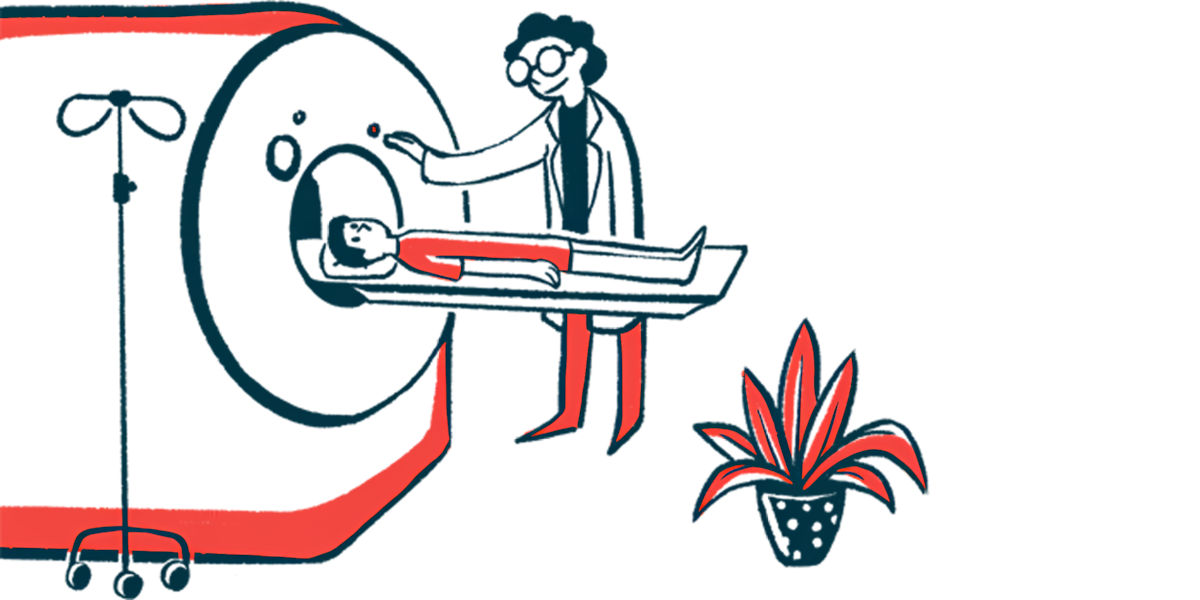
Using advanced diagnostic tools — such as different types of brain scans and methods that employ artificial intelligence (AI) — may help detect early signs of Parkinson’s that precede the onset of the disease’s motor symptoms, according to a new review study.
The researchers noted that employing these AI-based tools could lead to earlier diagnoses, and in turn, an earlier start to treatment, for people with Parkinson’s disease that’s still in the prodomal period — meaning, before its characteristic motor signs become noticeable.
“The aim of early detection of prodromal [Parkinson’s] is to allow us to include neuroprotective agents and lifestyle modifications that can slow progression of disease and improve long-term outcomes,” the researchers wrote.
According to the team, work in this area should focus on integrating imaging techniques with AI models and validating them to enhance diagnosis accuracy.
“By addressing these challenges, we can advance the early identification of [pre-diagnostic Parkinson’s], ultimately leading to better management and improved patient outcomes,” the researchers wrote.
Their study, “Multimodal diagnostic tools and advanced data models for detection of prodromal Parkinson’s disease: a scoping review,” was published in the journal BMC Medical Imaging.
Looking at the prodromal stage – before motor symptoms start
Parkinson’s is caused by the loss of dopaminergic neurons — the nerve cells that produce dopamine, a brain chemical messenger, particularly in a brain region called substantia nigra. The disease is characterized by motor symptoms that include tremors, slowed movements, rigidity, and postural instability.
The prodromal stage of Parkinson’s refers to early symptoms that appear before such motor signs are noted. Despite available diagnostic modalities, the identification of prodromal disease remains challenging, and most diagnostic tools have not been fully validated to screen patients at this very early stage of Parkinson’s, per the researchers.
To learn more, a team of scientists in Egypt and the U.S. conducted a review exploring the current diagnostic tools that are used to evaluate those suspected of having prodromal Parkinson’s. Their focus was on multimodal imaging analysis — a method that combines different types of brain scans, and AI-based approaches.
Overall, the analysis covered nine studies involving 567 participants with prodromal Parkinson’s and 35,643 controls. The ages of those taking part ranged from 63 to 77. The studies were conducted in different countries, including the U.S., the U.K., France, Germany, and China.
In five studies, advanced imaging techniques were used to detect early signs of Parkinson’s, particularly multimodal magnetic resonance imaging (MRI). This is the acquisition and analysis of multiple MRI sequences or contrasts to analyze different tissue properties.
Other techniques included diffusion tensor MRI, which allows the analysis of tissue microstructures, and neuromelanin-sensitive MRI designed to visualize and quantify neuromelanin, a pigment that is present in brain regions rich in dopaminergic neurons. Electrocardiogram (ECG), a noninvasive test to record the electrical activity of the heart, was also used.
According to researchers, “neuroimaging techniques … demonstrated potential in detecting early neurodegenerative changes.”
AI tools show promise, but validation, standardization is needed
The team noted that diffusion tensor imaging and neuromelanin-sensitive MRI were especially useful for showing changes in brain structure, while PET scans were used to measure problems with dopamine activity in the brain.
Additionally, four studies applied AI models to data from accelerometry and voice analysis. Accelerometry, which involves the use of wearable sensors to track movement, was used to detect subtle changes in movement speed and gait patterns, potential early indicators of Parkinson’s disease.
“Machine learning-based approaches … showed promise in improving diagnostic accuracy by identifying subtle physiological and behavioral markers indicative of prodromal [Parkinson’s],” the researchers wrote.
Across all included techniques, neuromelanin-sensitive MRI demonstrated the highest specificity (95%) for prodromal Parkinson’s, by effectively detecting signs of nerve cell degeneration in the substantia nigra. Meanwhile, ECG-based AI models yielded the highest sensitivity (84%) by identifying subtle heart issues in the prodromal stage.
Sensitivity refers to a test’s ability to correctly identify individuals who have the disease — known as true positives — while specificity refers to its ability to correctly identify individuals who do not have the disease, called true negatives.
This scoping review underscores the potential of advanced diagnostic modalities, particularly multimodal imaging and AI-based approaches, in enhancing the early detection of prodromal Parkinson’s disease. … Future research should prioritize the integration of multimodal imaging techniques with AI models to improve diagnostic accuracy and early detection.
Among patients included in all analyzed studies, several syndromes were observed, particularly idiopathic REM sleep behavior disorder. This sleep condition is characterized by dream enactment behavior in which people physically act out their dreams. It is a known risk factor for Parkinson’s.
Olfactory dysfunction, indicating an impaired sense of smell — considered an early potential indicator of Parkinson’s — was frequently assessed, the researchers noted. Some studies also evaluated mild motor impairments and autonomic dysfunction, which affects the autonomous nervous system that controls the body’s involuntary functions.
“This scoping review underscores the potential of advanced diagnostic modalities, particularly multimodal imaging and AI-based approaches, in enhancing the early detection of prodromal Parkinson’s disease,” the researchers wrote.
Noting that “these technologies demonstrate promising results,” the team reported that their clinical use “is hindered by limitations in validation and standardization.”
“Future research should prioritize the integration of multimodal imaging techniques with AI models to improve diagnostic accuracy and early detection,” the team concluded.