AI analysis helps identify molecular subtypes of Parkinson’s
Artificial intelligence uses patient-derived cells to help classify certain subtypes
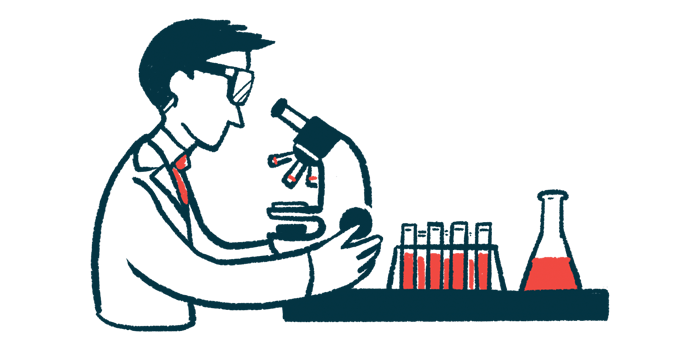
Using machine learning to analyze a patient-derived cell model may help to identify different molecular subtypes of Parkinson’s disease, a new study shows.
“Using a model of the patient’s own neurons, and combining this with large numbers of images, we generated an algorithm to classify certain subtypes – a powerful approach that could open the door to identifying disease subtypes in life,” Sonia Gandhi, MD, PhD, assistant research director at the Francis Crick Institute in London, and co-author of the study, said in a press release.
The study, “Prediction of mechanistic subtypes of Parkinson’s using patient-derived stem cell models,” was published in Nature Machine Intelligence.
Parkinson’s disease is highly variable; the specific symptoms and rate of disease progression can differ widely from person to person. This variability is presumably due to differences in how the disease occurs at the molecular and cellular level. However, these differences remain incompletely understood.
One of the main molecular drivers of Parkinson’s is thought to be abnormal aggregation or clumping of the protein alpha-synuclein. Dysfunction of mitochondria, the so-called powerhouses of the cell, also is thought to play a critical role in Parkinson’s.
“We understand many of the processes that are causing Parkinson’s in people’s brains. But, while they are alive, we have no way of knowing which mechanism is happening, and therefore can’t give precise treatments,” Gandhi said.
Four cellular models of Parkinson’s created
In this study, scientists used patient-derived cells to create four cellular models of Parkinson’s with different causes. Two of the models were characterized by alpha-synuclein clumping. One model harbored mutations that causes clumping and mimicked an agressive form of Parkinson’s. In the other model cells were treated with pre-existing clumps of the protein, which triggers more clumping inside the cell.
The other two models both were defined by reduced mitochondrial function. One model was treated with specific stressors that mimic what happens with certain mutations that disrupt the mitochondria. The other model was treated with a chemical to inhibit these cellular powerhouses, mimicking exposure to pesticides, a known cause of Parkinson’s disease.
Once the four different models were generated, the researchers conducted detailed imaging evaluations of the cells. They then used the imaging data to train a machine learning algorithm.
Machine learning, sometimes called artificial intelligence (AI), basically works by feeding a computer a set of data alongside a set of mathematical rules, called algorithms. Using the algorithms, the computer learns from the data — in this case, finding imaging features associated with each of the four cellular models.
“Now that we use more advanced image techniques, we generate vast quantities of data, much of which is discarded when we manually select a few features of interest,” said James Evans, a PhD student at the Crick Institute and co-author of the study.
Deep learning extracts ‘much more information’
“Using AI in this study enabled us to evaluate a larger number of cell features, and assess the importance of these features in discerning disease subtype. Using deep learning, we were able to extract much more information from our images than with conventional image analysis,” Evans said.
Once the computer was trained, the researchers fed it a set of new images to see whether it could accurately identify the molecular subtypes, as well as distinguishing them from healthy cells. Results showed it could identify the subtype with an average accuracy of about 95%. For some of the subtypes the accuracy was as high as 99%.
The researchers found that certain imaging features were crucial for the computer’s ability to differentiate between the different types. In particular, the amount of contact between mitochondria and lysosomes — molecular recycling centers that help to break down cellular waste, including damaged mitochondria — was crucial for distinguishing between the models driven by mitochondrial dysfunction of alpha-synuclein clumping.
These findings “demonstrate that the information independently contained within the mitochondria and lysosomes and the information contained within their interactions (for example, when lysosomes clear mitochondria) are both sufficient to predict—and are therefore likely to be biologically relevant in—the four mechanisms of disease described here,” the researchers wrote.
More research needed to expand molecular subtypes
The scientists noted that further work will be necessary to expand this approach beyond just the four molecular subtypes examined here. But, since these analyses were done using cells derived from patients, the researchers hope this technology could be used one day to help identify different molecular subtypes of Parkinson’s disease in living patients, which may help guide treatments.
“Taking this one step further, our platform would allow us to first test drugs in stem cell models, and predict whether a patient’s brain cells would be likely to respond to a drug, before enrolling into clinical trials,” Gandhi said. “The hope is that one day this could lead to fundamental changes in how we deliver personalised medicine.”