Machine Learning Can ID Patients by Voice, Distinguish Disease Stages
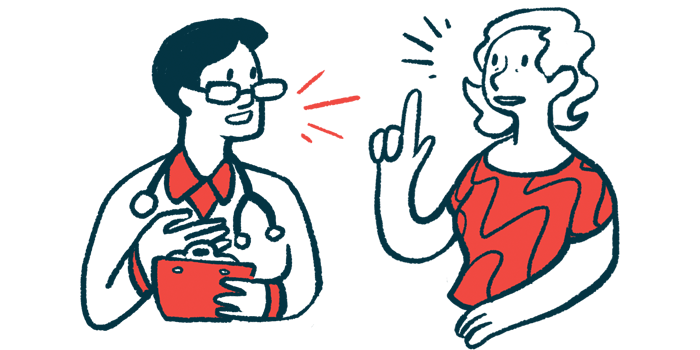
Machine learning — a form of artificial intelligence that allows a computer to adapt, analyze, and infer from patterns in data — can distinguish by voice people with Parkinson’s disease from those without it and track disease stages by continued changes in voice quality, a study reported.
Using speech recordings, researchers observed that patients’ voice first deviates from normal in early-stage Parkinson’s and further degrades as the disease progresses.
While levodopa, a mainstay Parkinson’s treatment, was able to improve speaking ability in people in middle and advanced disease stages, it did not restore voice.
These findings suggest that machine learning analyses can be a “near future … innovative” disease biomarker, and one “able to support the clinical management” of Parkinson’s, the researchers noted.
Reported to be the first such study in a large and varied patient group, “Voice in Parkinson’s disease: A machine learning study,” was published in Frontiers in Neurology.
Voice often changes in people with Parkinson’s as a result of diminishing muscle control. Their speech can become slurred, their voice quiet and monotone, or they can have difficulty pronouncing consonants and starting sentences.
A team of researchers in Italy and Jordan wondered if machine learning could improve how accurately doctors can assess voice changes in Parkinson’s patients. To do so, a computer would need to generate algorithms based on data from voice recordings.
Their study enrolled 115 Parkinson’s patients (mean age, 68.2), nearly half (57 people) with early-stage disease and never treated with levodopa, while the remaining 58 patients were middle-to-advanced stage and on long-term levodopa use. A total of 108 age-matched healthy individuals were included as controls.
Voice changes were noted in 97 (84%) patients, as indicated by a score equal to or greater than one on a sub-item of Unified Parkinson’s Disease Rating Scale (UPDRS) that assesses speech. These changes were evident in 68% of patients with early-stage disease and 100% of those in mid-to-advanced stages.
Recordings were used to determine whether machine learning could distinguish between people with and without Parkinson’s, and early-stage patients from those with more advanced disease.
Voice samples were recorded with patients — all native Italian speakers and non-smokers — saying the vowel “e” for at least five seconds. They then spoke a sentence using their usual voice intensity, pitch, and quality. Samples from seven patients had to be excluded due to corrupted files.
Machine learning algorithms were able to distinguish patients — including those at early disease stages —from healthy individuals using these recordings. They also distinguished between early-stage and more advanced patient groups, the researchers found.
“The application of machine learning analysis showed that voice is abnormal in PD [Parkinson’s disease] as demonstrated by high diagnostic accuracy in the discrimination of voices between PD patients” and healthy controls, they wrote.
Researchers also noted that 32% of early-stage patients — nearly one-third of this group — were not seen to “manifest a clinically overt voice impairment,” but were still distinguished from healthy controls using machine learning. “We speculate that the high accuracy in discriminating early-stage patients and [controls] would reflect the ability of machine learning to recognize subclinical voice impairment” in Parkinson’s, they wrote.
Levodopa’s effects on voice were examined by comparing patients using this treatment in either “on” or “off” times. “On” time occurs when levodopa is working well and symptoms are controlled; “off” time marks when treatment effects wear off and symptoms return before another dose of levodopa can be taken.
This treatment resulted in a significant clinical easing of voice impairments in people with mid-to-advanced disease as well as of motor control, the researchers noted. But levodopa’s use “improved voice less than other motor symptoms,” they wrote.
Levodopa also “failed to restore it [voice] as demonstrated by high diagnostic accuracy in the discrimination between” controls and patients in “on” levodopa states.
Machine learning algorithms, which used a “likelihood ratio” of voice impairment ranging from zero to one, were also able to distinguish between “on” and “off” state patients. The closer the likelihood ratio is to one, the greater the degree of voice impairment.
This ratio was also seen to correlate with clinical measures of disease severity — its duration, and patients’ overall degree of motor disability and cognitive impairment.
“Our study showed for the first time significant clinico-instrumental correlations: the higher LR [likelihood ratio] values attributed by machine learning, the longer the disease duration, the higher severity of motor symptoms, and finally the greater voice impairment in [Parkinson’s] patients,” the wrote.
“We demonstrated for the first time that the degree of voice changes in PD correlates with disease duration and severity,” the researchers concluded, adding that likelihood ratio values “can be considered reliable scores to express the complexity of voice impairment.”