Gait Difficulties, Tremors May Best Predict Future Diagnosis
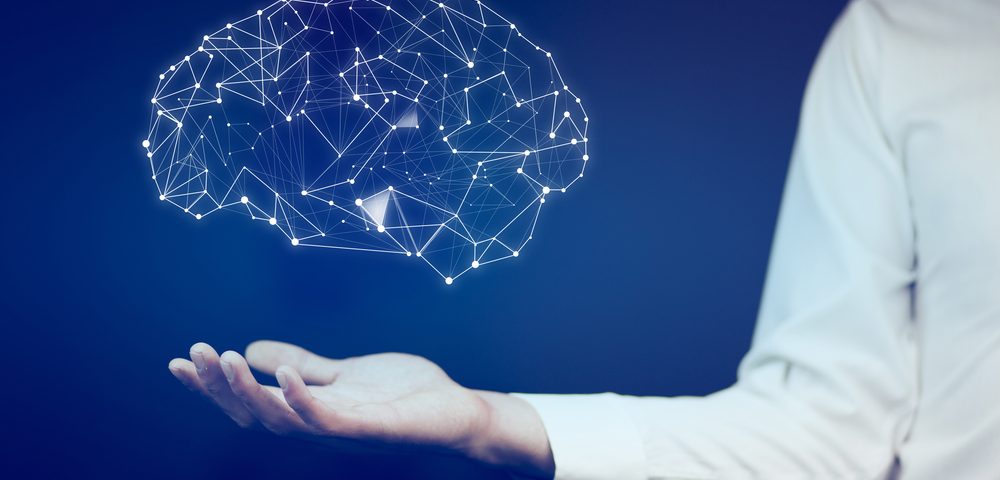
Computer algorithms used to analyze large medical databases found an early diagnosis of walking difficulties or tremors best predicted a future diagnosis of Parkinson’s disease, a study found.
The study, “Accelerating diagnosis of Parkinson’s disease through risk prediction,” was published in the journal BMC Neurology.
Evidence shows clinical symptoms of Parkinson’s disease emerge several years before diagnosis. These symptoms may include motor problems, such as tremors, walking (gait) difficulties, posture instability, and non-motor symptoms such as sleep deficits, loss of sense of smell, or depression and anxiety.
As such, early identification of these symptoms will enable a faster diagnosis, but also a better understanding of disease development, earlier treatment, and eligibility for clinical trials evaluating prevention strategies.
Medical records analysis using computer algorithms has demonstrated their real-world utility to predict eventual Parkinson’s diagnosis. However, many of these attempts used data closer to the time of diagnosis and may be influenced by a clinician’s suspicion of Parkinson’s. Furthermore, the clinical features used to train these algorithms may not represent what a physician would see in real-world practice.
Now, a team of researchers based at the Harvard Medical School in Boston, Massachusetts designed a new computer algorithm to analyze two large health-record databases, They sought to help identify which individuals progress to Parkinson’s using features well in advance of diagnosis that are more easily identifiable by clinicians in real-time.
“Ultimately, accurate, prospective identification of high-risk individuals would allow for earlier diagnosis, intervention, and more effective large-scale evaluation of potential therapeutics,” the team wrote.
The first source used was the Partners Healthcare Research Patient Data Registry, a database of electronic medical records (EMR) from about six million individuals in Massachusetts. The second data source was de-identified administrative claims from a large private insurance company representing more than 75 million members with ZIP codes outside Massachusetts as not to overlap with the first dataset.
Minimum age of 50 at diagnosis (baseline) was used to exclude those with disease driven by genetics. Participants also were required to have at least two years of claims data before diagnosis and two years after to capture the prodromal (pre-diagnostic) period and track progression.
From the EMR database, 3,251 Parkinson’s patients were selected, of which 58.5% were men, with a mean age at first symptom observation of 63.15 and a mean age of diagnosis of 72.48. A total of 18,851 age- and sex-matched non-Parkinson’s controls were included.
The claims database analysis included 5,131 patients, of which 61.4% were men, with a mean age at first symptom observation of 69.01 and a mean age of diagnosis of 73.70. A total of 23,085 age- and sex-matched controls were included in this group.
Two models were employed: a standard statistical regression model evaluating individual occurrences of disease features and a deep learning neural network model to investigate a patient’s sequence of claims over time.
Both models were created for each time point examined corresponding to different prediction windows, including 0, 15, 30, 45, 75, 90, 180, 270, 360 (one year), 450, 640, and 720 days (two years) before Parkinson’s diagnosis. For each of these time points, a two-year observation window preceding the specific time point was used. The team then sequentially compared different time periods before the Parkinson’s diagnosis date.
Analysis results
The analysis first found a significant rise in prediction accuracy as the window size was reduced, which reached a maximum immediately before diagnosis. The accuracy of both regression and neural network models converged closer to the diagnostic date. In contrast, prediction accuracy using earlier disease features was resolved only by the neural network model.
Both prediction models suggested a pre-diagnostic window during which motor symptoms were reported, but a diagnosis had not been made.
The dominant features in this pre-diagnostic window included a diagnosis of abnormal gait, as well as a diagnosis of tremor disorders, such as abnormal involuntary movements and essential tremors, characterized by the rhythmic shaking of the hands, head, trunk, voice, or legs.
Other features were well-known, prodromal features, including depression and constipation, and other less-traditional features such as fatigue, pain, and type 2 diabetes.
Next, the rates of different diagnoses relative to the Parkinson’s diagnosis date corresponding to gait disorders, tremor disorders, and constipation (a known prodromal symptom) were used to better characterize their predictive ability.
With constipation, there were elevated rates of diagnosis that rose steadily before and after diagnosis. In contrast, gait and tremor disorders among Parkinson’s cases slowly diverged from controls until a large spike was seen about one year prior to the Parkinson’s diagnosis date.
Based on the importance of a gait and tremor diagnosis in the pre-diagnostic models, the team constructed models for three groups: those with a first diagnosis of gait or tremor; those diagnosed with tremor only; and patients with gait difficulties only.
The neural network model analyzing the claims database found those diagnosed with a gait problem or tremor generated an area under the curve (AUC) of 0.874. Of note, an AUC assesses accuracy, with an AUC of 0.8 to 0.9 considered excellent. In contrast, using either gait or tremor alone, the AUCs were less than 0.8. The standard regression model using claims data for gait or tremor generated an AUC of 0.803, whereas the same model using EMR data gave an AUC of 0.804.
An examination of the most robust model for the average days-in-advance to predict Parkinson’s for those who went on to receive a diagnosis found the average was in excess of 300 days. That indicated “model performance is not dominated by individuals who immediately go on to develop [Parkinson’s] after a gait or tremor diagnosis, and that among this selective [group], early diagnosis is feasible,” the researchers wrote.
Finally, while constipation is a known early pre-diagnostic symptom, it was less useful at predicting who will progress to Parkinson’s from gait or tremor.
In conclusion, the study showed that “gait and tremor disorders represent anomalous patterns of diagnosis or differential diagnosis, suggesting the existence of a population of [Parkinson’s] patients whose diagnoses can be accelerated,” the researchers wrote.
“These findings enable more nuanced predictive algorithms that better resemble the patient populations that physicians are likely to encounter in practice and potentially those likely to respond to different interventions,” they added.