AI Algorithm May Help Monitor Parkinson’s Severity Remotely, Study Suggests
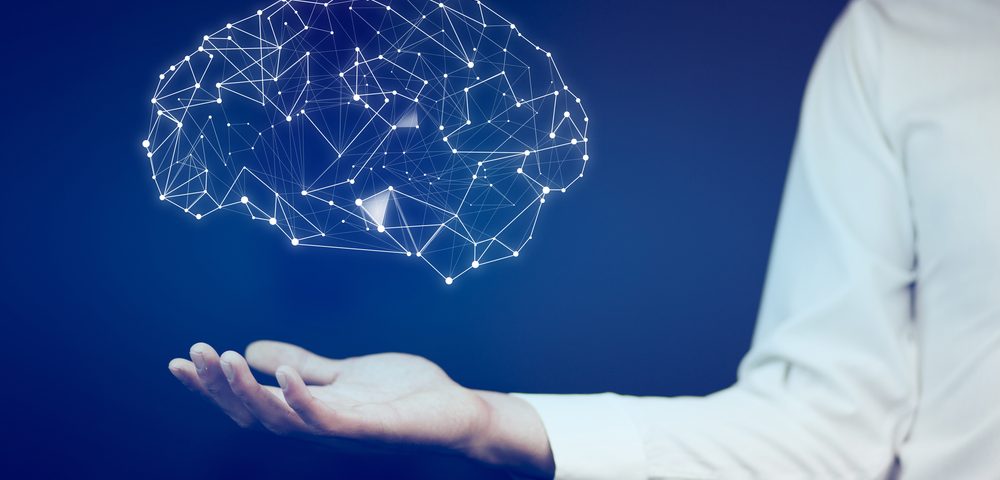
Estimating the severity of Parkinson’s disease may be possible by remotely measuring and analyzing physical activity as patients’ motor impairment increases, research led by scientists at IBM Research suggests.
The method uses an artificial intelligence (AI) algorithm that examines movement data sent from wrist-worn accelerometers. It can be used to monitor patients in either a clinic setting, or in more natural environments, such as a patient’s home.
The study, “Using an unbiased symbolic movement representation to characterize Parkinson’s disease states,” was published in the journal Nature Scientific Reports.
Parkinson’s disease patients typically are assessed through a combination of patient-reported information and scales such as the Movement Disorder Society’s Unified Parkinson’s Disease Rating Scale (MDS-UPDRS).
These assessments help determine not only the severity of disease, but also enable physicians to adjust dopaminergic medications to a patient’s needs. Too much of these medications can cause a faster habituation and lead to a faster wearing-off, while too little may increase the risk of falls.
However, most scales used to monitor patients are subjective. With the MDS-UPDRS test, for example, patients are asked to perform a series of tasks, which are rated subjectively by clinicians based on their quality, amplitude, or frequency.
With the development of wearable technologies, patients now can be monitored continuously for movements, which could provide more accurate (less subjective) data to follow disease progression and responses to medication.
So, scientists at IBM Research, in collaboration with the Pfizer Innovation Research Lab, Boston University’s Spivack Center for Clinical & Translational Neuroscience and Tufts Medical Center’s Department of Neurology sought to investigate whether these wearable sensors could be coupled with an AI algorithm to estimate the severity of Parkinson’s symptoms.
Drawing an analogy to human speech, the researchers broke human movement into a sequence of “syllables,” whose patterns vary by both external factors and by one’s internal neurological state.
Human movement consists of a series of discrete, or stereotyped movements that are linked together in predictable ways, like the way we move our arms back and forth when walking. But these movements are affected in people with neurodegenerative conditions, and patterns can be compared to establish healthy ones from disordered ones.
The study included 45 healthy participants (mean age 44) and 35 Parkinson’s patients (mean 69) who were asked to wear accelerometers (devices that measure movement) and perform a series of tasks involving walking and hand movements.
To assess the algorithm’s effectiveness at estimating the amount of gait impairment and the severity of patients’ Parkinson’s symptoms, all participants also were assessed by a neurologist with a standard MDS-UPDRS test.
Researchers also tested their algorithm in an additional 26 Parkinson’s patients who wore a GeneActiv device, which is used to monitor physical activity, at home to determine whether data extracted in more natural environments could provide similar information as that taken in a clinic.
An AI algorithm then analyzed each participant’s movement patterns, learning healthy patterns from disordered ones. After training, the algorithm was able to estimate the severity of symptoms in patients with high accuracy, the team noted.
This study was part of IBM Research’s BlueSky Project with Pfizer, which seeks to use AI to improve accuracy in clinical trials. The data used for this study came from three prior BlueSky Project studies that had collected wearable sensor data from three groups: people diagnosed with Parkinson’s and undergoing a standard neurological exam; healthy participants undergoing the same procedure; and people with Parkinson’s moving freely at home.
The IBM team hopes that access to data from both clinical and at-home settings will enable neurologists to evaluate those with Parkinson’s more accurately.
Other potential advantages of this technique include speed and continuity.
Less than 10 minutes of activity, on average, were needed to create stable estimates. The wearable sensor allows continuous evaluation of a user’s neurological state, which is particularly important since Parkinson’s symptoms are known to fluctuate throughout the day.
Finally, the technique’s use of remote sensing fits in well with the growing need for telemedicine during the COVID-19 pandemic, especially with Parkinson’s patients, who are more vulnerable to the infection and more often housebound.
“The proposed method differentiates motor impairment accompanying various [Parkinson’s] related states both in clinical settings when scripted activities are performed, and in unconstrained everyday life,” the investigators wrote.
The team also noted that this method may have applications beyond Parkinson’s.
“The proposed model was explicitly tested on movement differences associated with [Parkinson’s] but we see no reasons why it should not be generalizable to detecting other neurological states with characteristic movement signatures,” they concluded.